Real-world data sets often contain anomalies or outlier data points. The cause of anomalies may be data corruption as well as experimental or human error. Anomalies can impact the performance of the model, so, if you want to train a robust data science model, you need to make sure the data set is free from any anomalies. That’s what anomaly detection does.
5 Anomaly Detection Algorithm Techniques to Know
- Isolation forest
- Local outlier factor
- Robust covariance
- One-class support vector machine (SVM)
- One-class SVM with stochastic gradient descent (SGD)
In this article, we will discuss five anomaly detection techniques and compare their performance for a random sample of data.
What Is Anomaly Detection?
Anomalies are data points that stand out from other data points in the data set and don’t confirm the normal behavior in the data. These data points or observations deviate from the data set’s normal behavioral patterns.
Anomaly detection is an unsupervised data processing technique to detect anomalies from the data set. An anomaly can be broadly classified into different categories:
- Outliers: Short/small anomalous patterns that appear in a non-systematic way in data collection.
- Change in events: Systematic or sudden change from the previous normal behavior.
- Drifts: Slow, unidirectional long-term change in the data.
Anomaly detection is very useful to detect fraudulent transactions, disease detection or handle any case studies with high-class imbalance. Anomaly detection techniques can be used to build more robust data science models.
How Does Anomaly Detection Work?
Simple statistical techniques such as mean, median and quantiles can be used to detect univariate anomaly feature values in the data set. Various data visualization and exploratory data analysis techniques can also be used to detect anomalies.
Anomaly Detection Algorithms to Know
We will discuss some unsupervised machine learning algorithms to detect anomalies, and further compare their performance for a random sample data set.
- Isolation forest
- Local outlier factor
- Robust covariance
- One-Class support vector machine (SVM)
- One-Class SVM with stochastic gradient descent (SGD)
1. Isolation Forest
Isolation forest is an unsupervised anomaly detection algorithm that uses a random forest algorithm, or decision trees, under the hood to detect outliers in the data set. The algorithm tries to split or divide the data points such that each observation gets isolated from the others.
Usually, the anomalies lie away from the cluster of data points, so it’s easier to isolate the anomalies from the regular data points.
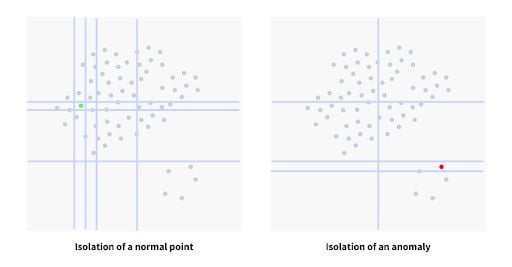
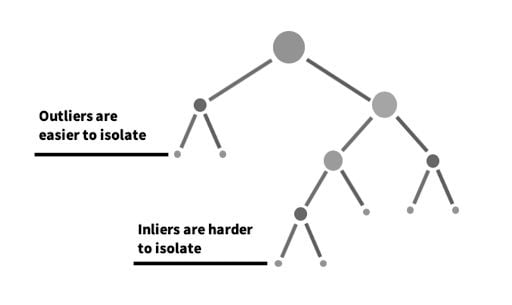
In the images above, the regular data points require a comparatively larger number of partitions than an anomaly data point.
The anomaly score is computed for all the data points and any points where the anomaly score is greater than the threshold value can be considered as anomalies.
2. Local Outlier Factor
Local outlier factor is another anomaly detection technique that takes the density of data points into consideration to decide whether a point is an anomaly or not. The local outlier factor computes an anomaly score that measures how isolated the point is with respect to the surrounding neighborhood. It takes into account the local and global density to compute the anomaly score.
3. Robust Covariance
For gaussian independent features, simple statistical techniques can be employed to detect anomalies in the data set. For a Gaussian/normal distribution, the data points lying away from third deviation can be considered as anomalies.
If every feature in a data set is Gaussian, then the statistical approach can be generalized by defining an elliptical hypersphere that covers most of the regular data points. The data points that lie away from the hypersphere can be considered as anomalies.
4. One-Class Support Vector Machine (SVM)
A regular support vector machine algorithm tries to find a hyperplane that best separates the two classes of data points. In an SVM that has one class of data points, the task is to predict a hypersphere that separates the cluster of data points from the anomalies.
5. One-Class SVM With Stochastic Gradient Descent (SGD)
This approach solves the linear one-class SVM using stochastic gradient descent. The implementation is meant to be used with a kernel approximation technique to obtain results similar to sklearn.svm.OneClassSVM which uses a Gaussian kernel by default.
Which Anomaly Detection Algorithm Should You Use?
The five anomalies detection are trained on two sets of sample data sets, row 1 and row 2.
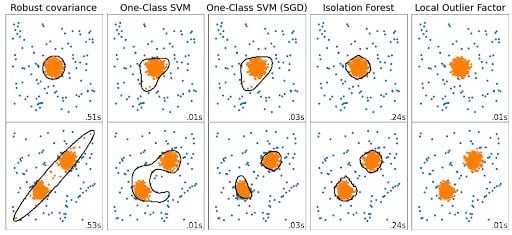
One-class SVM tends to overfit a bit, whereas the other algorithms perform well with the sample data set.
Advantages of Using an Anomaly Detection Algorithm
Anomaly detection algorithms are very useful for fraud detection or disease detection case studies where the distribution of the target class is highly imbalanced. Anomaly detection algorithms are also to further improve the performance of the model by removing the anomalies from the training sample.
Apart from the above-discussed machine learning algorithms, data scientists can always employ advanced statistical techniques to handle the anomalies.