The Z-test is a statistical hypothesis test used to determine where the distribution of the test statistic we are measuring, like the mean, is part of the normal distribution.
There are multiple types of Z-tests, however, we’ll focus on the easiest and most well known one, the one sample mean test. This is used to determine if the difference between the mean of a sample and the mean of a population is statistically significant.
What Is a Z-Test?
A Z-test is a type of statistical hypothesis test where the test-statistic follows a normal distribution.
The name Z-test comes from the Z-score of the normal distribution. This is a measure of how many standard deviations away a raw score or sample statistics is from the populations’ mean.
Z-tests are the most common statistical tests conducted in fields such as healthcare and data science. Therefore, it’s an essential concept to understand.
Requirements for a Z-Test
In order to conduct a Z-test, your statistics need to meet a few requirements, including:
- A Sample size that’s greater than 30. This is because we want to ensure our sample mean comes from a distribution that is normal. As stated by the central limit theorem, any distribution can be approximated as normally distributed if it contains more than 30 data points.
- The standard deviation and mean of the population is known.
- The sample data is collected/acquired randomly.
Z-Test Steps
There are four steps to complete a Z-test. Let’s examine each one.
4 Steps to a Z-Test
- State the null hypothesis.
- State the alternate hypothesis.
- Choose your critical value.
- Calculate your Z-test statistics.
1. State the Null Hypothesis
The first step in a Z-test is to state the null hypothesis, H_0. This what you believe to be true from the population, which could be the mean of the population, μ_0:

2. State the Alternate Hypothesis
Next, state the alternate hypothesis, H_1. This is what you observe from your sample. If the sample mean is different from the population’s mean, then we say the mean is not equal to μ_0:

3. Choose Your Critical Value
Then, choose your critical value, α, which determines whether you accept or reject the null hypothesis. Typically for a Z-test we would use a statistical significance of 5 percent which is z = +/- 1.96
standard deviations from the population’s mean in the normal distribution:
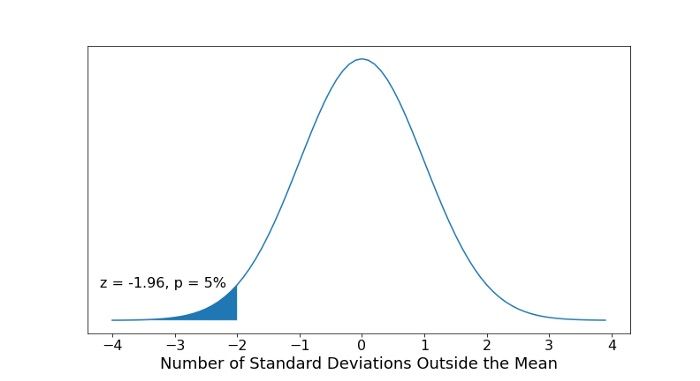
This critical value is based on confidence intervals.
4. Calculate Your Z-Test Statistic
Compute the Z-test Statistic using the sample mean, μ_1, the population mean, μ_0, the number of data points in the sample, n and the population’s standard deviation, σ:

If the test statistic is greater (or lower depending on the test we are conducting) than the critical value, then the alternate hypothesis is true because the sample’s mean is statistically significant enough from the population mean.
Another way to think about this is if the sample mean is so far away from the population mean, the alternate hypothesis has to be true or the sample is a complete anomaly.
Z-Test Example
Let’s go through an example to fully understand the one-sample mean Z-test.
A school says that its pupils are, on average, smarter than other schools. It takes a sample of 50 students whose average IQ measures to be 110. The population, or the rest of the schools, has an average IQ of 100 and standard deviation of 20. Is the school’s claim correct?
The null and alternate hypotheses are:

Where we are saying that our sample, the school, has a higher mean IQ than the population mean.
Now, this is what’s called a right-sided, one-tailed test as our sample mean is greater than the population’s mean. So, choosing a critical value of 5 percent, which equals a Z-score of 1.96, we can only reject the null hypothesis if our Z-test statistic is greater than 1.96.
If the school claimed its students’ IQs were an average of 90, then we would use a left-tailed test, as shown in the figure above. We would then only reject the null hypothesis if our Z-test statistic is less than -1.96.
Computing our Z-test statistic, we see:

Therefore, we have sufficient evidence to reject the null hypothesis, and the school’s claim is right.
Hope you enjoyed this article on Z-tests. In this post, we only addressed the most simple case, the one-sample mean test. However, there are other types of tests, but they all follow the same process just with some small nuances.