The future we’ve seen in science fiction movies is here. From virtual reality to functional gadgets, AI has invaded our lives in a multitude of ways. AI is playing an increasingly important role in the evolving tech landscape. The technology propelling it might seem like magic, but it’s not. It’s just mathematics.
3 Math Concepts to Learn for AI
- Linear algebra: This field allows you to abstract data and models and is critical to building machine learning models.
- Calculus: This field is critical to modeling and making predictions with AI.
- Probability: Understanding probability theory offers tools to deal with uncertainty and is crucial to building robots and other innovations.
The ideas behind thinking machines and the possibility to mimic human behavior are done with the help of mathematical concepts.
Artificial intelligence and mathematics are the two branches from the same tree. And if you want to thrive in your AI career, you need to study mathematics. Being a fan of science fiction won’t be enough. Be friends with mathematics, and it will rock your world.
As a mathematician and a fan of AI, I want to share the magical connection between those two fields. Let’s dive deeper into the fascinating realm.
How Is Math and AI Connected?
Artificial intelligence problems constitute two general categories: Search problems and representation problems. Following them are interconnected models and tools like rules, frames, logics and nets. All of them are mathematical topics.
The primary purpose of AI is to create an acceptable model for human understanding. And these models can be prepared with the ideas and strategies from various branches of mathematics.
Consider self-driving cars. Their goal is to recognize objects and people in video images. Math powers these cars in the form of minimization procedures and backpropagation. Math helps AI scientists solve challenging, deep abstract problems using traditional methods and techniques that have been known for hundreds of years.
Math for AI Explained
Behind all significant advances in technology, there is mathematics. The concepts of linear algebra, calculus, game theory, probability, statistics, advanced logistic regressions and gradient descent are all major underpinnings of data science.
Math helps in understanding logical reasoning and attention to detail. It enhances your abilities to think under pressure and increases your mental endurance. Mathematical concepts provide the real solution to hypothetical or virtual problems. It’s about structure, developing principles that remain true even if you make any alteration in the components.
For now, let’s focus on the three main branches of mathematics that will help lead to a thriving career in AI. These include: Linear algebra, calculus and probability.
Linear Algebra
Linear algebra is the field of applied mathematics that AI experts can’t live without. You will never become a good AI specialist without mastering this field. Linear algebra helps in generating new ideas, that’s why it is a must-learn thing for AI scientists and researchers. You can abstract data and models using linear algebra concepts like scalars, vectors, tensors, matrices, sets and sequences, topology, game theory, graph theory, functions, linear transformations, eigenvalues and eigenvectors.
Vectors
In linear programming, vectors are used to deal with inequalities and systems of equations for notational conveniences. AI scientists use different techniques of vectors to solve problems of regression, clustering, speech recognition and machine translation. The concepts are also used to store the internal representations of AI models like linear classifiers and deep learning networks.
Matrix Theory
The concept of matrix theory is used in the study of neural networks. When you form artificial neurons in three layers — input, hidden and output — you can make non-linear hypotheses in a neural network.
AI scientists classify the neural networks from their quantity of hidden layers and the way they connect. It’s not all that different from a real neuron. A neural network can be formed by those artificial neurons, and it took about 20 years to discover this.
Eigenvalues and Eigenvectors
The science behind search engine ranking is based on mathematical science. Page rank, which is the very groundwork of Google, is based on the mathematical perspective. Page rank is the algorithm, originally formulated by Larry Page and Sergey Brin in their research paper “The Anatomy of a Large-Scale Hyper-textual Web Search Engine.” They applied the basic concepts of principal eigenvalues and eigenvectors, which has been around for hundreds of years, to power this breakthrough.
Robot crawlers first retrieve the web pages, and then index and catalog them by assigning page rank values. The credibility of each page depends on the number of links to that page.
The rank r(P)
of a given page P
is assumed as:
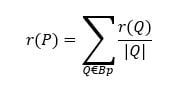
Where:
Bp
= all pages pointing toP
.|Q|
= number of outlinks fromQ
.P
is the matrix with the terms:
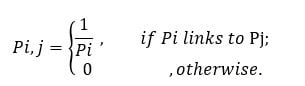
To find the convergence and convergence rates, the matrix P is adjusted. When the row Google matrix P reaches the sum to 1, then it is called a row stochastic matrix. The page rank iteration represents the evolution of a Markov chain in which the web directed graph is represented as a transition probability matrix P. It shows the probability of a random surfer at each of the three pages at any point in time.
First, a binary adjacency matrix is created to signify the link structure, then it is transformed into a probability matrix by normalizing it.
To compute the page rank, it’s essential to solve an eigenvector problem for the linear system:
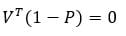
The eigenvalues of Stochastic matrix P can be assumed as 1> λ1 ≥ λ2 ≥ … ≥ λn, and V1, V2, V3, ……
, Vn
be the corresponding eigenvectors.
After the process of convergence, the dominant eigenvalue of matrix P should be λ=1
to satisfy the equation:
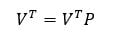
With:

Which is the steady state distribution of the Markov Model. This is how Google automatically characterizes the page rank value of each site.
Calculus
Differential calculus, multivariate calculus, integral calculus, error minimization and optimization via gradient descent, limits and advanced logistic regressions are all the concepts used in mathematical modeling. A well-designed mathematical model is used in the biomedical sciences to simulate complex biological processes of human health and diseases with high fidelity.
In silico modeling, which is the application of AI approaches in biomedicine, is a fully automated model that does not require human samples, crude animal tests, clinical trials or laboratory equipment. A differential mathematical equation is used in the model to test new mechanistic hypotheses and evaluate novel therapeutic targets. It is the most inexpensive and convenient way to study human physiology, drug responses, and diseases way more accurately by manipulating mathematical model parameters.
Probability
There are a lot of abstract problems in the AI world. You may experience uncertainty and stochasticity in many forms. Probability theory offers tools to deal with uncertainty. The concepts of probability are used to analyze the frequency of an event.
Let’s consider a robot. A robot can only move forward for a certain number of seconds, but not a certain distance. To make the robot go forward, scientists use mathematics in its programming. Discrete random variables, continuous random variables, Bayes formula and normalization are some concepts of probability that are used in robotics navigation and locomotion along with other concepts of linear algebra.
Advantages of Learning Math for AI
Whether you want to pursue a career as a machine learning engineer, a data scientist or a robotic scientist, you need to excel in mathematics. Mathematics can enhance analytical thinking, a skill that’s vital in AI. People often think AI is magic, but it isn’t. It’s mathematics that creates the magic behind these inventions. To lead in today’s AI-driven world, you need to master mathematical concepts like linear algebra, calculus and probability.