If you’re a Pandora listener and want a quick rush of nostalgia, play Thumbprint Radio. It’s a playlist of every song you’ve ever approved with a thumbs up. It will make you feel old, young, and, if you’re anything like me, astounded by where life has taken you. Bill Withers. Willy Nelson. Belle & Sebastian. Antibalas. Missy Elliott. Could these artists’ works possibly be approved by the same person?
Much of the content we digest every day is served to us by recommendation engines, and customization through explicit signals, such as a thumbs-up endorsement or five-star rating, is part of how these engines learn about us. But only a small part, said Michael Scharge, author of the September release Recommendation Engines, published by MIT Press.
Whether you listen to Pandora, Spotify, YouTube or Apple Music, your listening habits and social networks are feeding these algorithms information about your tastes and preferences. And recommendation engines are not just dialing in your musical DNA, of course. Whether it’s the Top Row picks of a Netflix homepage or a line of suggested apparel at Neiman Marcus or Barney’s New York, recommendation engines have become adept at harvesting data to tailor their suggestions to our online profiles.
But from a design perspective, what makes a recommender system good? How can the organization of an interface hone in on what we already like, yet still help us discover new dimensions of ourselves? How can the system avoid feeling overly prescriptive or becoming an echo chamber? How can it filter out the occasionally misleading artifacts of our online behavior?
Lauren Pufpaf, who spent 25 years as a DJ spinning downtempo and house records in clubs from London to New York, has given these questions careful consideration. She is now the chief operating officer of Feed.fm, a B2B music service that works with fitness companies and niche apps to develop stations optimized for people’s workouts.
In some ways, she said, a good recommendation engine is akin to a good DJ; it works within a pre-established framework of choices but can improvise on the fly, using human curation to subtly tweak the mix.
“You pick a set of music, and then go into an environment with a bunch of people and see what they’re vibing to and then alter it real time,” Pufpaf said. “So it’s that idea of creating a set, but also having the skill and enough music to be able to be dynamic with your choices.”
7 Tips for Designing An Effective Recommendation System
- Don’t let algorithms pigeonhole you.
- Give users an array of choices.
- Use content and collaboration filters to boost engagement.
- Be transparent in your recommendations.
- Balance what you know people like with opportunities to branch out.
- Master your metadata.
- Customize the UX with sharing, surveys and ratings.
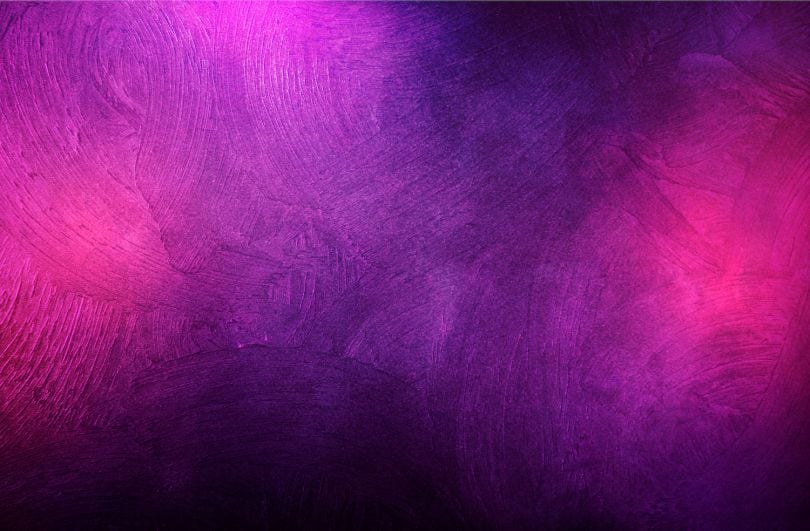
Don’t Let Algorithms Pigeonhole You
While Feed.fm leverages machine learning algorithms on the front end to sift through metadata — instrumentation, beats per minute, a happiness quotient — a team of professionally trained musicians and producers shape the sequence and emotional arc within a station and introduce fresh choices to listeners.
“We’re really interested in freshness. So on a monthly basis, we’ll pull things out and save them for later. Because even if you really like Dua Lipa, you don’t want to hear five of her songs in a given playlist,” Pufpaf said.
“Even if you really like Dua Lipa, you don’t want to hear five of her songs in a given playlist.”
Owners of streaming services like Apple Music have also recognized the importance of applying a human curation filter, she said, working with DJs and celebrity tastemakers to sift through algorithmically generated selections to create customized playlists.
“If you just let the algorithm run, I think what people are starting to feel is this idea of an echo chamber, where I’m just getting the same stuff all the time, right? Because it matches things so well,” Pufpaf said.
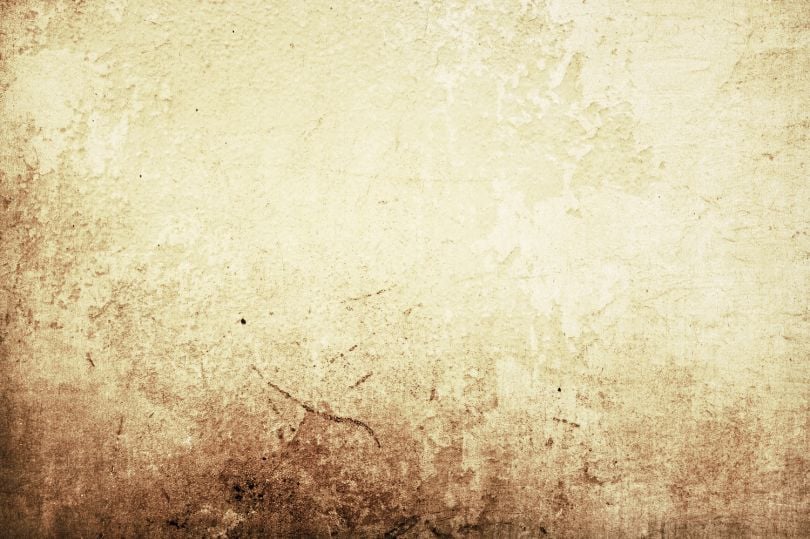
Give Users an Array of Choices
In his book, Scharge points out that “the essential function of recommender systems is mathematically predicting personal preference.” Their effectiveness, he argues, hinges on choice. Surfacing an array of choices, not just one or two, is a strategy companies like Spotify, Netflix, TikTok and Amazon all practice to learn about their customers and strengthen the predictive power of their algorithms.
“If you look at Spotify’s discover feature, which has done remarkably, astonishingly well, it doesn’t recommend songs. It recommends playlists. The unit of analysis for Spotify is not a genre of music, but what’s on a playlist. So the diversity, the portfolio aspect, is built in. And that’s not a subtle distinction. That’s an enormous distinction,” Scharge said.
“The unit of analysis for Spotify is not a genre of music, but what’s on a playlist.”
Currently, Spotify bundles music and podcast packages by playlists that offer subscribers a range of options, from “Made for You” and “Mood” playlists to artist’s picks and selections popular among users' followers.
“So you’re the algorithm, you’re Spotify,” he explained. “What should we push? Should we push the artist? Should we push the tempo? Should we push the contrast with the song that preceded it in the playlist? Or should we push the greatest level of commonality? You admire this artist. Well, maybe we should let you know about that artist’s playlist. And that’s what Spotify does.”
Pufpaf said user choice is also at the heart of Feed.fm’s recommender strategy. The company creates stations for customers that are individually tailored to specific workouts and the brand guidelines of their clients — companies like Mirror, Tonal, Life Fitness and Nautilus. A mid-intensity cardio class will offer different pre-set options than a yoga class, but still allow room for users to pick from a sampling of styles and genres.
“We’re going to give you the choice of 10 different soundtracks for a strength workout,” she said. “You might say, ‘I’m in the mood for hip hop.’ We’ll give you hip hop that is the right fit for that particular workout.”
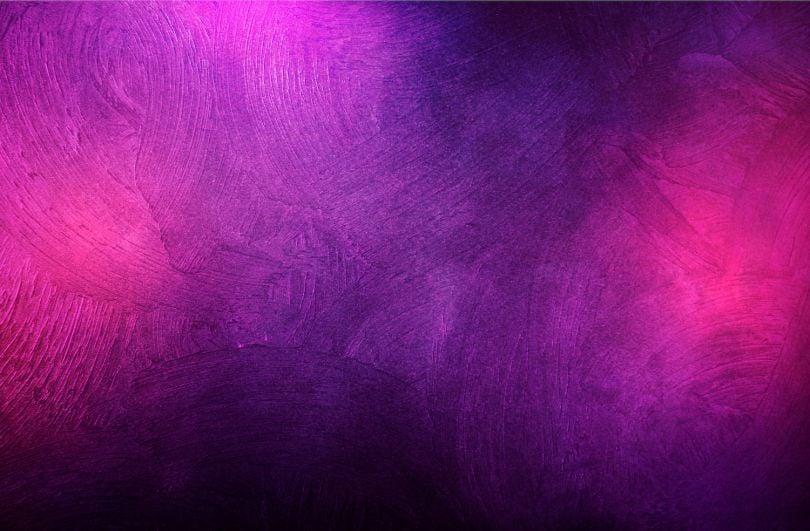
Use Content and Collaboration Filters to Boost Engagement
The best recommendation engines perform meta-level pattern recognition, not only looking for similarities, but predicting the kind of similarities that will appeal to users.
Some suggestions are based on the content itself, Scharge said. In a movie, this could be the genre, the actors who appear in it, the budget, the awards it was nominated for or any number of tagged features. Ensembles of algorithms study “the pattern of these patterns” to learn which are most predictive of users’ behavior. Does a user like The Matrix because it is sci-fi or cyberpunk? Or do they just have a Keanu Reeves fetish?
Other recommendation engines make suggestions based on collaborative filters, the psychographic data and listening habits of similar groups of people.
“Do you listen to Spotify for five for 20 minutes at a time, the same time every morning? Or do you listen to it for 90 minutes or two hours a day uninterrupted?” Scharge asked. “You can be damn sure that, if you fall into the two hours a day uninterrupted category, you’re being lumped in with a different group of ‘people like you.’”
By mining massive data sets, the best recommendation engines leverage both approaches — and complex mathematical formulas involving categorization and regression analysis — to encourage users to interact with media.
“There’s a very, very thin line between recommenders and social media,” Scharge said. “Because remember, recommenders are about content. And people like you. And once you break the wall by sharing, it becomes content, people like you, people you share with, and people who share with you.”
In a two-part blog series co-written by Justin Basilico, the research and engineering director at Netflix, and Xavier Amatrian, the co-founder of Curai and a former Netflix research director, the authors note that “75 percent of what people watch is from some sort of recommendation.” Interface personalization, they say, is central to Netflix’s recommendation system and is based on “the way we select rows, how we determine what items to include in them, and in what order to place those items.”
The genre of each row, the titles that appear on it and how titles are ranked are designed to maximize customer satisfaction. Recently viewed movies, ratings, the interests of others in a household and a person’s social circle all factor into which recommendations get surfaced and the order in which they appear.
“Our business objective is to maximize member satisfaction and month-to-month subscription retention, which correlates well with maximizing consumption of video content,” the authors write. “We therefore optimize our algorithms to give the highest scores to titles that a member is most likely to play and enjoy.”
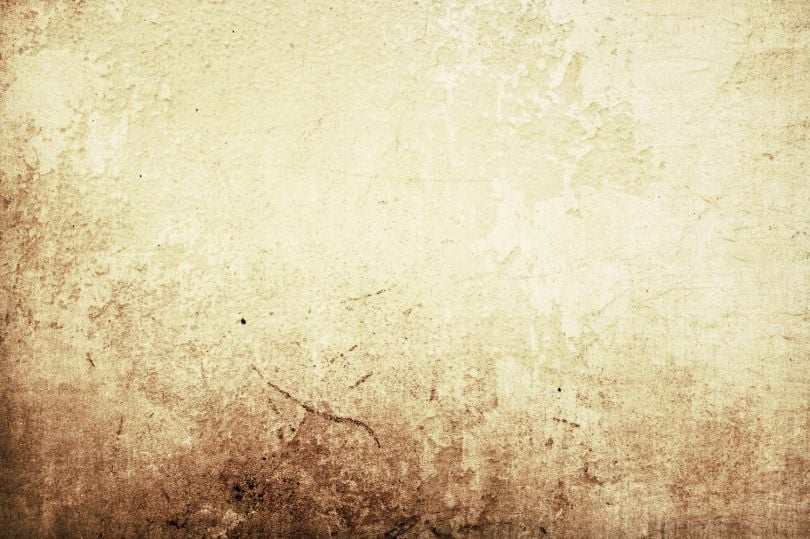
Be Transparent in Your Recommendations
User awareness is important too. Signaling to users why you are feeding them particular recommendations, Scharge said, is crucial to building trust and, ultimately, boosting engagement. A Spotify feature allowing users to follow artists they admire, and listen to their playlists, is one example of this sort of transparency.
“Because even if you don’t like that music, you’ll understand the reason why it was put in your discover queue,” he said. “Eddie Van Halen is gone. But he put his playlist on Spotify. And these are his favorite songs; you’re getting them because you like Eddie Van Halen.”
Transparency happens in subtler ways too. Explanations on Spotify’s homepage reveal why recommendations were chosen. Maybe they are popular with listeners of a particular artist. Maybe they reflect a member’s recent listening history. Perhaps they are well-liked by fans of a user’s favorite artists. Gray category labels on the interface clarify these distinctions: “Popular with listeners of Sugar Calling,” or “For fans of Kacey Musgraves.”
Pandora, whose team of musicologists has categorized 450 musical attributes as part of the Music Genome Project, can all but end the debate on why John Lennon and Paul McCartney’s songs sound so different. From instrumentation and lyrics to vocal performance and melodic structure, the artists have distinct sonic signatures. Paul has broader vocal range, John is a howler. Paul likes eighth-note shuffles, John likes waltz time. Their Pandora bios tease out these predilections.
“I would love the ability to personalize my recommendation algorithm through some interface on Spotify, particularly, like a slider of discoverability versus familiarity.”
Netflix goes even further, revealing its best guess of how well a movie matches your personal taste, and labeling movies based on their popularity, ratings and those in your social circle who have watched them.
But for all these cues, it is still something of mystery as to how algorithms sort, filter and score selections. Pufpaf said she would like to see a UX feature that lets users adjust the balance of the algorithms feeding them suggestions.
“I would love the ability to personalize my recommendation algorithm through some interface on Spotify, particularly, like a slider of discoverability versus familiarity,” Pufpaf said. “Right now, I don’t know what their percentage is. But it feels very low on the discoverability score. Every person is different, but, specifically, for my job, I would like new things served to me. So if I could say, ‘I want 70 percent new, 30 percent familiar,’ that would be an incredible next step.”
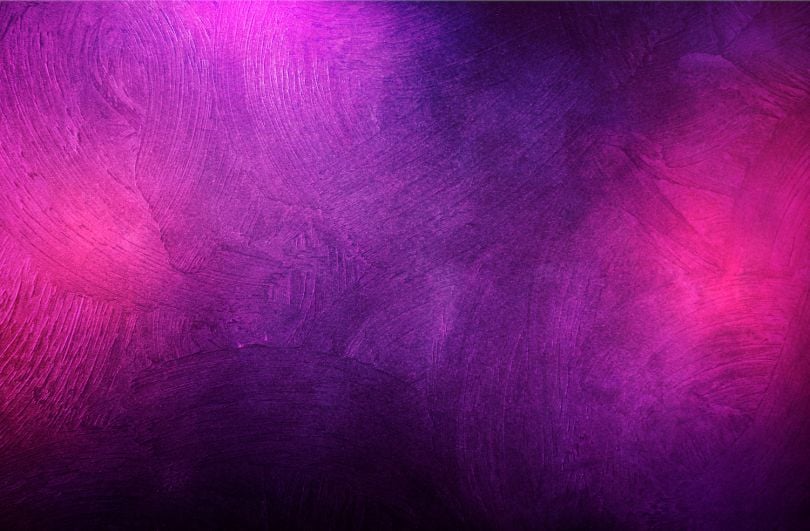
Balance the ‘Exploitation Versus Exploration’ Ratio
Companies are already working backstage to address the desire for diversity, according to Scharge. He said so-called greedy algorithms, or multi-arm bandit algorithms, are designed to optimize the balance between the familiarity of users’ recommendations and their diversity and novelty. Finding the preferred ratio, a dilemma some machine learning experts refer to as exploitation versus exploration, is what these algorithms attempt to do by analyzing the strength and frequency of the the signals a user is sending.
“If I’m listening to The Who, The Beatles, Stevie Wonder, The Byrds and The Beach Boys, I’m probably going to be getting ’60s and ’70s pop, right. And they’ll experiment. Should it be the American California sound? Should they throw in a Bjork? Clearly, I’m interested in understanding the lyrics. So would they put Mason William’s Classical Gas in there? Because it was a big hit in the sixties? I don’t know,” Scharge said.
What he does know is that algorithms are working to figure out the answer: That is, how much tolerance users have for new discoveries. In what domains are they willing to stretch themselves? What are their boundaries?
“That’s exactly the kind of issue that a recommender is going to confront: explore versus exploit,” Scharge said. “Should I go with something familiar from the ’60s with words? Or should I go with a really, really successful song from the ’60s that’s instrumental. And if they listen to Classical Gas without skipping it, I get to diversify. I’ve learned something as a recommender. If they skip over it, stick to words. Maybe slip Sinatra in there doing New York, New York.”
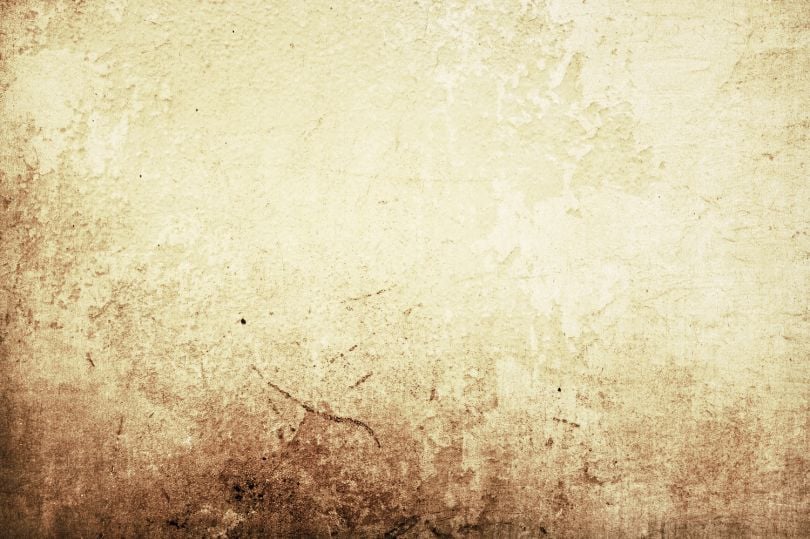
Master Your Metadata
Borrowing a catchphrase from Basilico and Amatrian in his article, “Everything Is a Recommendation,” Tim Mullaney, writing for MIT Technology Review, notes that online recommendation engines are becoming subtler and more prolific, thanks to better tagging technologies and larger data sets.
“One example is how recommendations may show up as auto-completing search results,” he writes. “After a shopper at Jenson USA’s bike shop enters the first two letters of a search for ‘full-face helmet,’ the recommendation system displays a list of helmets in an order based on the customer’s profile.”
TikTok is another prime example of tagging sophistication. Recommendations that appear in the “For You” tab are highly customized, derived from a classification system optimized for virality. Whether a video is forwarded, and whether people adopt its moves and music — that is, whether it is meme-worthy— are key factors in determining how it is circulated to users.
“TikTok can identify dancing cats and dancing dogs,” Scharge said. “That’s how much progress we’ve made on recommendation engines. We can recommend dancing cats and dogs and pets, according to your taste in music. We couldn’t do that 10 years ago. And TikTok is worth billions because it can do exactly that.”
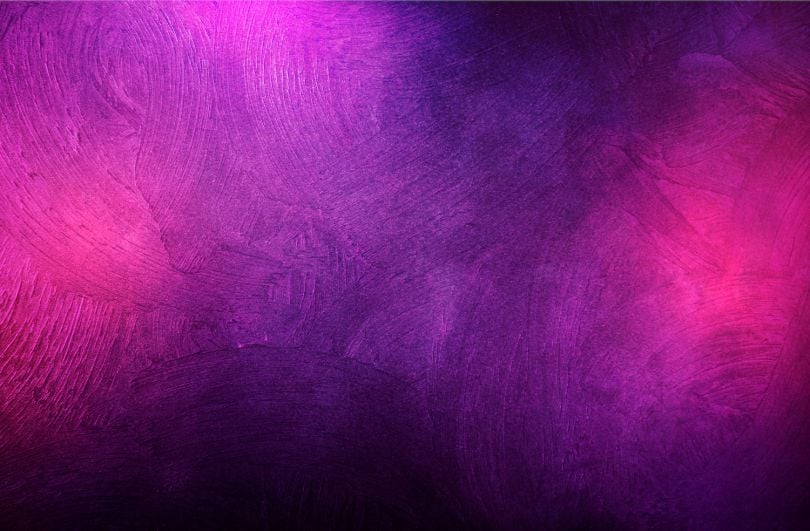
Customize the User Experience With Sharing, Surveys and Ratings
TikTok’s recommendation engine, he told me, is the unofficial gold standard when it comes to fostering user empowerment. Contrary to the judgment of critics like Cornell University student Niko Nguyen who charge that the dance-fueled, video-barrage can inspire addiction, Scharge sees TikTok’s influence as largely benign — even inspirational.
“Users can be inspired by a funny video and make their own and have a million people follow it,” he said. “Or a million people seek to replicate it. My God. Andy Warhol, that’s old hat. Fifteen minutes? Screw that. Fifteen million.”
More often, though, recommendation engines empower users in modest ways. Feed.fm, like countless platforms, offers thumbs up and thumbs down buttons, options to favorite songs with heart icons, and a feature that allows users to skip up to six songs an hour. Onboarding quizzes train the company’s algorithms to the artists, songs and genres users prefer.
But the bulk of the data, as with most recommendation engines, comes from less overt user behavior.
“So did you go all the way through the song or did you skip part of it? Did you drop out of the workout? The interesting thing about the implicit data is there’s just a lot more of it for us to analyze,” Pufpaf said.
Ultimately, though, all metrics lead back to a company’s vision and KPIs. For the most enlightened tech firms, Scharge said, the aim of a recommendation engine is not just to help people connect with their current or past selves, it’s to lead them toward self-actualization, to help them become who they wish to be.
“Spotify wants to be the soundtrack of your life, right? And that’s why they’re moving into the podcast phase,” he said. “Mark my words, they are going to have a mindfulness and stress relief and feel-good-about-yourself program ... if they aren’t already starting it.”