While ChatGPT is producing benefits for people, the popular generative AI tool also carries risks that government and industry should partner to manage.
5 Ways to Minimize the Risk of Generative AI
- Build a catalog of AI applications for your business.
- Establish a governance structure for AI.
- Evaluate risks, including copyright infringement and customer privacy.
- Require that humans oversee and validate the models’ output.
- Track compliance.
While governments and industry groups are sorting out how exactly to do that, however, companies need to take action now to mitigate the risks inherent in generative AI tools and to ensure that their use of those tools remains safe, legal and responsible.
Specific risks of generative AI vary depending on whether you are using ChatGPT or an equivalent system directly, such as for composing marketing emails; using applications enabled by large language models such as GitHub Copilot, a tool to help developers write code; or using large language models for in-house development of an application such as a support chatbot in-house.
Regardless of the specifics, the broad areas of risk and risk mitigation remain the same. Here are five steps companies can take today to minimize the risks that arise from using these powerful new generative AI tools.
Establish an AI Catalog
The key to minimizing generative AI risk is knowing where and how your data science and product groups use these technologies today, and where and how your teams propose to use them in the future. The most effective way of doing so is by building a catalog of AI applications and models and establishing a well-defined process for managing the lifecycle of generative AI applications.
For example, your teams should be tagging all the generative AI applications they are using with corresponding copyright policies, the sensitivity of the data used in the model, potential exposure of confidential data, whether they are subject to regulations like the European Union’s proposed AI Act and other key risk factors.
Alongside the AI catalog, the organization should define clear procedures for proposing, approving and implementing new uses of these technologies. Here is a sample process workflow:
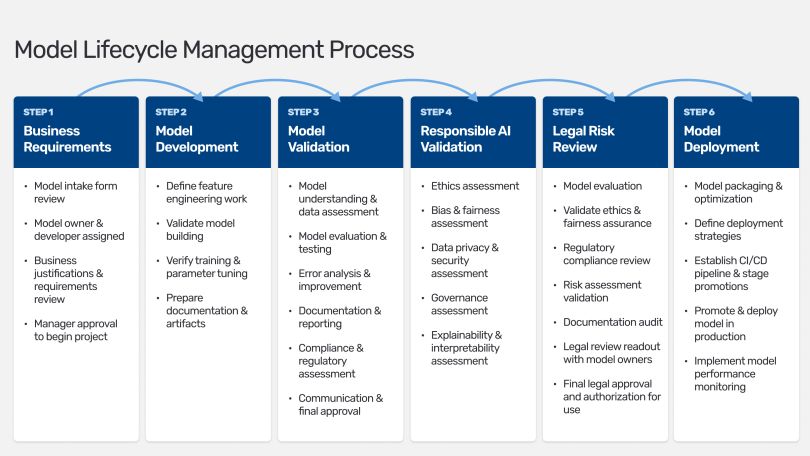
Companies also should agree on a standardized model risk checklist that includes all the pertinent risk- and governance-related information for their models. A sample model risk checklist could include:
- Business unit
- Model risk rating
- Contains copyright
- Market jurisdiction
- California Privacy Rights Act (CPRA)
- GDPR PII
- EU AI Act risk level
- EU AI Act risk level restriction(s)
- EU AI Act Exemption
- Generative AI
- Compute intensity rating
- ESG risk rating — energy consumption
Establish a Governance Structure
As with managing other kinds of risks, a governance structure is essential for managing generative AI risks on an ongoing basis. A governance structure includes:
- The individuals and groups responsible for governing the use of generative AI
- Processes and workflows to propose, enable and monitor the use of generative AI
- Clear, documented policies governing generative AI technology
- Tools for managing the use of generative AI
Frequently, the individuals and groups responsible are a combination of enterprise risk management, legal, information security and data science. Processes and workflows are also fairly similar across organizations, often including intake workflows, legal approval, data approval and so on. Upcoming regulations on AI are expected to further systemize these workflows.
Each enterprise also needs to formalize policies that govern the use of generative AI so that teams can determine how to best leverage these technologies while minimizing risks. Finally, tools such as the AI catalog and generative AI APIs enable the implementation of these governance policies and processes, providing the information, documentation and audit trails required for governance.
Evaluate Your Risks
Bias is a risk with any AI system that is basing decisions on historical data: Making creditworthiness decisions using data that reflect past economic and racial disparities is very likely to perpetuate those disparities.
Similarly, asking a generative AI image-creation tool to produce a picture of a CEO is likely to result in an image of a white male when the tool’s training data reflect a preponderance of this demographic in corporate leadership positions.
What is unique about generative AI is that the training datasets for these tools are often massive and frequently unknown. For example, OpenAI trained its GPT-3 model on half a trillion tokens, and it declined to disclose details of the training for the successor GPT-4 model. These two factors make it highly problematic to identify, understand and mitigate potential biases baked into the models’ training data.
Additional unique aspects of risk arising from generative AI use include the potential to inadvertently use copyrighted material or leak proprietary or customer information and general uncertainty around output quality.
As a result, organizations must vet every application of generative AI along these dimensions, using approval processes like those described above, before releasing an application into the world. Moreover, since an intelligent AI system will consist of multiple AI components, companies must assess the potentially varying characteristics of these components along these dimensions before using them.
Require Human Oversight and Rigorous Validation
Because the outputs of generative AI models are unpredictable and potentially fabricated (i.e., hallucinations), rigorous validation and human oversight are needed to build confidence in the quality of the models’ output.
If your organization is using third-party end-to-end applications powered by generative AI, the application provider may provide validation information. However, currently this kind of documentation is scarce and may not apply to your use case. Therefore, users need to perform independent validation for their specific use case.
Finally, if your company is developing an application using third-party foundation models for commercial purposes, the onus to thoroughly validate and document the performance of the generative AI application rests with your organization.
Track Compliance
Work with your legal team to track regulations to ensure that your company’s use of generative AI complies with existing and imminent regulatory requirements such as the European Union’s GDPR regulation governing data privacy and the AI Act.
For example, as currently proposed, the EU AI Act would require extensive documentation and review of different aspects of so-called high-risk AI systems. Gathering all the required information on all the AI systems in production within an organization will be time-consuming and laborious. Therefore assessing the impact of the EU AI Act on your business is critical to managing generative AI risk and ensuring against business disruption when the act’s provisions come into force.
We are still in the early days of understanding all the potential beneficial applications for generative AI, let alone the immense potential of these applications to rewrite whole businesses. But it’s not too early for organizations to assess the reputational, legal and financial risks inherent to these same tools. The time for companies to take steps to mitigate those risks is now, before any harm is done.