It would seem to go against Marketing 101, but is it possible that, in certain instances, strong sales and positive consumer feedback for a new product can be… bad?
Absolutely, if the consumers ponying up for that new product also have a demonstrated history of favoring items that fail. Those shoppers are, as marketing expert Eric Anderson and his colleagues described in a seminal 2015 research paper, “harbingers of failure.”
As it turns out, the people who purchased Diet Crystal Pepsi were more likely to have purchased Frito Lay Lemonade, researchers found. (If neither rings a bell, well, exactly.) And they kept purchasing — while they could, that is — only furthering the mirage of a supportive market.
“A one-time purchase of Diet Crystal Pepsi is partially informative about a consumer’s preferences,” the researchers wrote. “However, a consumer who repeatedly purchased Diet Crystal Pepsi is even more likely to have unusual preferences, and is more likely than other customers to choose other new products that will fail in the future.”
The research contradicted conventional marketing models, like the Bass diffusion model, that correlate strong early sales with a greater chance of long-term success. It showed, in short, that a quick start off the mark does not a marathon runner make.
The research also converged with the early days of retail’s embrace of big data. The authors analyzed two large samples of data from a national drugstore: one data set of individual customer transaction data, spanning more than 10 million transactions made using customer-loyalty cards over two years; and a sample of aggregate store-level transaction data, spanning 111 store locations in 14 states over more than six years.
The academic analysis may have been more post hoc than what we often see in contemporary business architectures — ingesting data to warehouses and lakes, then pipelined for analysis or reporting from business intelligence or data science teams — but it was nonetheless a triumph of data mining, or using large volumes of data to unearth significant patterns and anomalies.
Top Benefits of Data Mining in Marketing
- Basket Analysis: What is it? Uncovering which products or services are often purchased together. How does it use data mining? Association rule learning.
- Product Recommendation: What is it? Tailoring product suggestions to individual users based on data. How does it use data mining? Association rule learning, along with techniques like collaborative filtering and content-based filtering.
- Customer Segmentation: What is it? Subgrouping customers or clients into subsets based on common characteristics and habits. How does it use data mining? Cluster analysis.
- Customer Lifetime Value: What is it? Quantifying how much money a customer is likely to generate for a company. How does it use data mining? Decision trees and boosting.
- Churn Prediction: What is it? Quantifying the likelihood a customer will stop doing business with a company. How does it use data mining? Classification and regression.
Since the publication of Harbingers of Failure, the authors have been honored for the paper’s “significant, long-term contribution” to marketing. It even spawned a 2019 sequel (there are entire ZIP codes that are harbingers of product failure, and residents are more likely to donate to unsuccessful congressional candidates, too). And, of course, the proliferation of big data has only intensified, which would seemingly prompt companies to implement the paper’s findings and steer clear of such harbingers as much as possible. But that hasn’t exactly happened.
“I keep running into people who know about this … but firms are really struggling to figure out what to do about it,” Anderson told Built In. “Most of them have a shockingly narrow view of the world.”
Most companies only buy data that it perceives as directly relevant, Anderson said. A cosmetics company, say, will probably purchase beauty-related data from a consumer packaged goods market research firm like Nielsen or IRI, but that probably doesn’t tell them who’s drinking the 2021 version of Frito Lay Lemonade.
Cross-category data can be hard to come by because data sets are often rigidly segregated. Anderson recalled how, while at Ocean Spray, his team would purchase data that only covered red drinks. It provided eyes on the cranberry juice world, “but you didn’t see anything related to other beverages,” said Anderson. “It wasn’t the beverage database, it was the red drink database.”
Anderson believes that many-tentacled retailers and e-commerce sites like Amazon and Walmart are best poised to implement his research, in part because they possess reams of sales data across so many categories.
The harbinger effect, however, is tangentially related to several examples of how data mining and marketing do intersect in the real world. It is, in effect, a bizarro-world version of basket analysis — the technique marketers use to tease out how consumers who like product X also tend to have affinity for product Y, or put them together in their basket.
It could also have implications for customer lifetime value, which tells companies which clients drive the most value — and should therefore be catered to most. (Harbinger customers, interestingly, are not bad for retailers. They can be counted on to keep shopping, just not for the “right” items.) Also, designating customers as red flags for failure is essentially a novel twist on the de rigueur use of customer segmentation, or dividing consumers into subsets based on some characteristics or habits.
Below are a few examples of data mining in marketing with real-world track records of success. Consider them harbingers of marketing success.
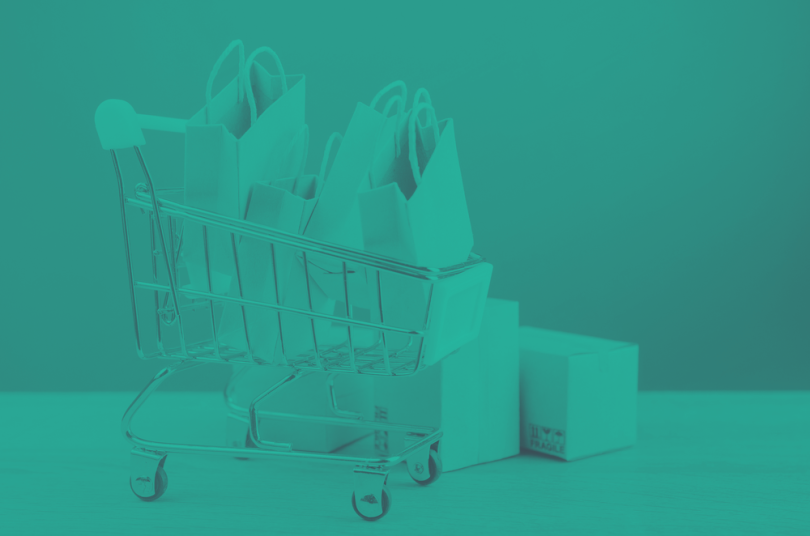
Basket Analysis
The story of beer and diapers has been around for decades. A data-savvy retailer supposedly crunched the numbers and discovered that shoppers often bought the two unrelated products together at the same time of day. It was, the story goes, young fathers who, when out on late-night diaper runs, awarded themselves with a six-pack, either as a treat or out of a two-birds-with-one-stone sense of efficiency. The story is almost certainly apocryphal, but it’s a helpful illustration of how purchase patterns have implications for how companies choose to cross-promote and target their marketing efforts.
For example, analytics firm Quantzig in 2019 integrated the disparate data sources of a European food retailer, then applied association rule learning to maintain a dashboard of real-time product-bundling recommendations. Those bundling suggestions netted a nearly 300 percent increase on advertising returns, according to the firm.
Instacart, not surprisingly, is also illustrative of basket analysis in practice. The company mines its masses of data to uncover affinities, which it occasionally shares with the public. Vegetable buyers tend to be “heavy meal preppers” who map out their weekly meal plans, often with tortillas, cucumbers and watermelons, while fruit buyers are more of the snacking type, also frequently grabbing yogurt and hummus, according to a recent Instacart blog post.
Incidentally, the grocery data set released by Instacart in 2017 — the largest of its kind — is an excellent resource for data-driven marketers looking to grok the data science behind consumer habit prediction.
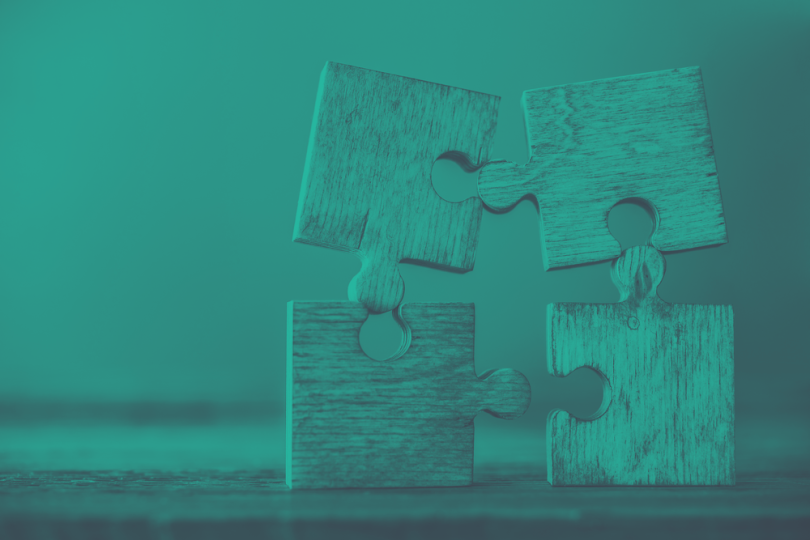
Product Recommendation
Those examples come from the packaged goods world, but the same idea of course is central to contemporary e-commerce, where the concept is known more simply as product recommendation.
Etsy, for example, has more than 80 million items for sale, so it operates a sophisticated recommendation system that helps prevent paradox-of-choice paralysis, Etsy chief technology officer Mike Fisher told the Wall Street Journal last year. The system has evolved over the years into a natural-language-processing framework that incorporates past searches and purchases — “billions of historical data points,” according to the Journal. Recent research published by Etsy data scientists also proposed mining recent user activity data to drive “within-session” personalization of attribute preferences, like color, size and material options.
Streaming platforms like Netflix and Spotify — which uses a hybrid of collaborative filtering and content-based recommendation — have also shown how data mining for sophisticated recommendation-driven engagement can drive success.
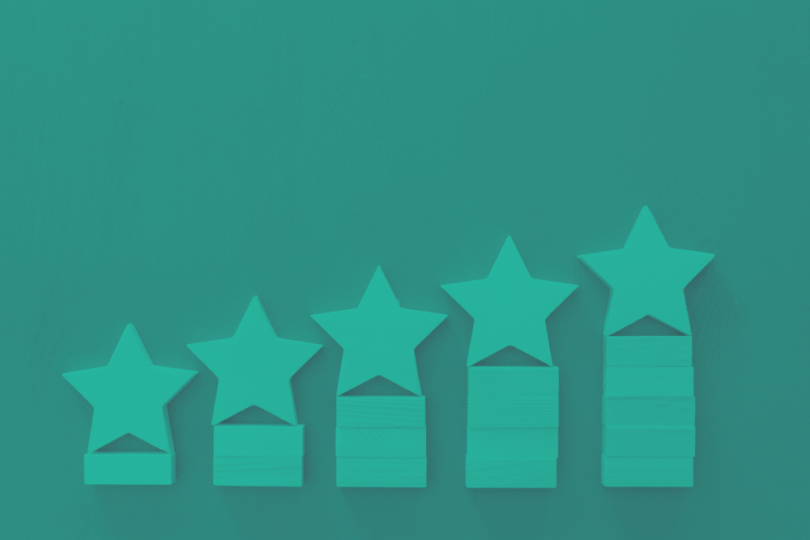
Customer Lifetime Value
All people may be created equal, but not all customers are equally deserving of a company’s efforts. That was the central point made by Peter Fader, the marketing expert who pioneered the concept of customer lifetime value (CLV) — a projection of how much profit a customer will generate — when he spoke to Built In earlier this year. Companies shouldn’t exhaust budgets “trying to turn ugly ducklings into beautiful swans,” but rather steer promotional outreach based on how much a customer is worth, he said.
Today, that means complex machine learning rooted in data mining — whether it’s the gradient-boosting decision trees of Cars.com or the neural networks that help power CLV software provider Retina. One of the most sophisticated examples, according to Fader, is game publisher Electronic Arts, which updates CLV estimates daily based on gamer behavior data. EA went from spending 22 percent of revenue on marketing down to less than 12 percent after it initiated updates to its CLV model, Zach Anderson, former chief analytics officer at EA, said on the Customer Equity Accelerator podcast in 2018.
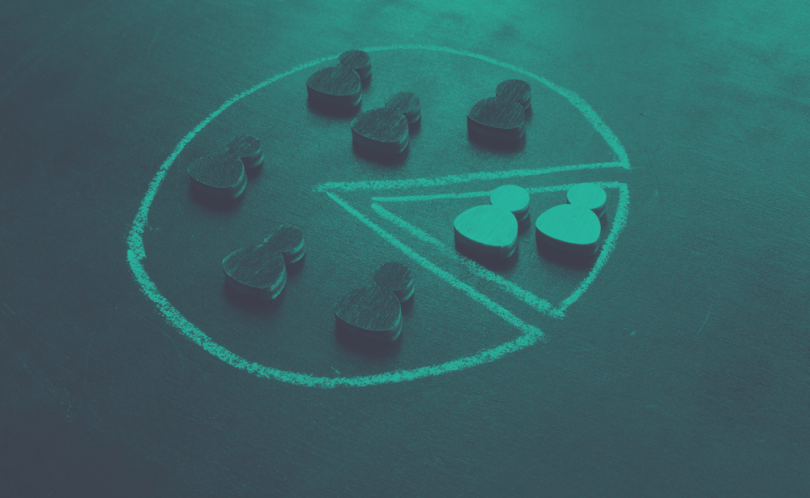
Customer Segmentation
The benefit of dividing a company’s customer base into distinct subgroups is probably obvious: Marketers can tailor messaging and promotions based on how that specific group interacts with the brand. But segmentation can’t be arbitrary, and data mining allows for meaningful customer segmentation.
The data mining method called cluster analysis is a go-to technique in marketing analytics. Data teams use cluster techniques, such as k-means clustering, to determine which data points are near or far apart in a distribution — or what users are similar and dissimilar. From that analysis, relevant customer personas emerge. The spectrum in, for instance, this post that analyzes an e-commerce data set spans six clusters/personas, ranges from “medium income, low annual spend” to “high income, high annual spend” to “very high income, high annual spend.”
Another cluster-based data mining technique is latent class cluster analysis (LCCA), which allows modelers to build segments using data beyond non-numerical data. Dallas-area analytics firm Decision Analyst used LCCA for a customer segmentation job when a client was introducing a new appliance to market, building clusters of like-minded panel responses to determine how best to position the new wares.
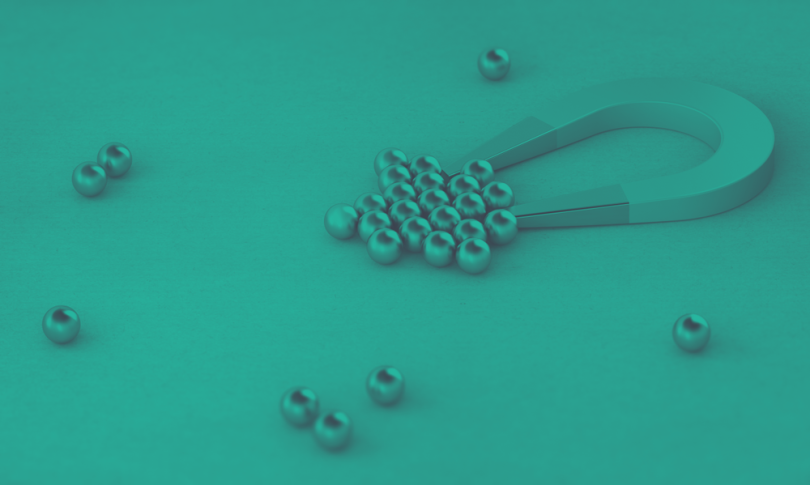
Churn Prediction
Marketing point of fact: It’s cheaper to keep a customer than it is to acquire a new one. Churn prediction is the attempt to gauge the likelihood that a client will cancel or not renew a service, a signal that marketers can then use to hopefully intercept that turnover before it happens.
Data mining techniques like humble regression analysis and classification are traditional cornerstones of churn prediction. (The classic Telco churn data set is generally used for classification.)
As quantitative researcher and Built In expert contributor Sadrach Pierre noted earlier this month, companies can now use Python libraries like Streamlit to build classification/churn models that are fronted with intuitive interfaces. There’s also a growing ecosystem of machine learning-enhanced churn prediction tools that, thanks to the high number of data points they process, can provide churn scores farther out.
“You can figure out how you’re going to tackle churn with your product shifts, and how you’re going to make it easier for the customer to work with you,” Kristen Hayer, founder of consulting firm The Success League, told Built In in May. “It changes the conversation because it gives you enough time to actually plan.”