Ever dealt with duplicate data? Like trying to figure out if Mary Smith from London, UK, is the same person as Mary Smyth from London, Canada?
This is an entity resolution challenge, and you're not alone.
Thousands of businesses face this issue daily. It’s such a widespread problem that Amazon launched a machine-learning-powered entity resolution tool to help companies match and link data for better customer insights.
If this sounds familiar, this article is for you. I’ll explain what entity resolution is, why it’s important, and which technologies are available for businesses, including SMEs that can’t afford million-dollar solutions.
Entity Resolution Defined
Entity resolution is the process of reviewing database records to determine where duplicate records exist and should be merged into one. This activity is essential both for ensuring your data can provide actionable insights and also for complying with data privacy regulations.
What Is Entity Resolution?
Entity resolution has traditionally been a data science topic, but it’s now gaining attention from businesses as they recognize the impact of duplicated, scattered, and siloed data on customer insights, operational efficiency, and decision-making. In fact, with GDPR laws, companies are now required to make sure they’re not accidentally sending unsolicited emails to customers who have explicitly requested to unsubscribe. To meet these and other data regulations, companies now must review their data to ensure records are clean, reliable and accurate.
For example, take the customer Mary Smith, whose records have multiple variations in the same ecosystem. If a company has data streaming in from multiple sources like third-party social media apps or service providers, Mary’s records are duplicated and each is treated as an individual record instead of being consolidated. If a company accidentally sends a confidential email to the wrong Mary, they risk fines and penalties.
Until recently, these issues would go unnoticed. With the emergence of fraud, stringent laws, and now AI, companies need reliable data more than ever.
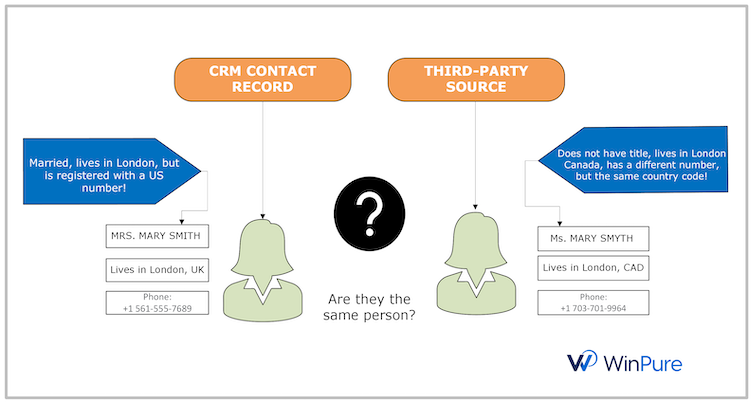
Entity resolution, therefore, is the process of correcting and consolidating these different variations of an entity — defined as a person or an object in a database — to create a unified profile. It helps answer a key question: Do these differing records belong to one person or more? Knowing the answer to this will not only save the company from unnecessary risks but also empower them with better customer insights.
Be warned, though, that entity resolution is a highly technical challenge. In the table below, you can see how different factors cause records to be the same. These aren’t just simple typos that you can fix with Excel. Such variations require advanced match logic to resolve.
For example, they may be the result of cultural differences like Aleksandr versus Alexander. Or in the case of Chris Johnson, this could be the name of a company or the name of an individual. The third column shows examples of different approaches to determining how these identities can be matched and checked.
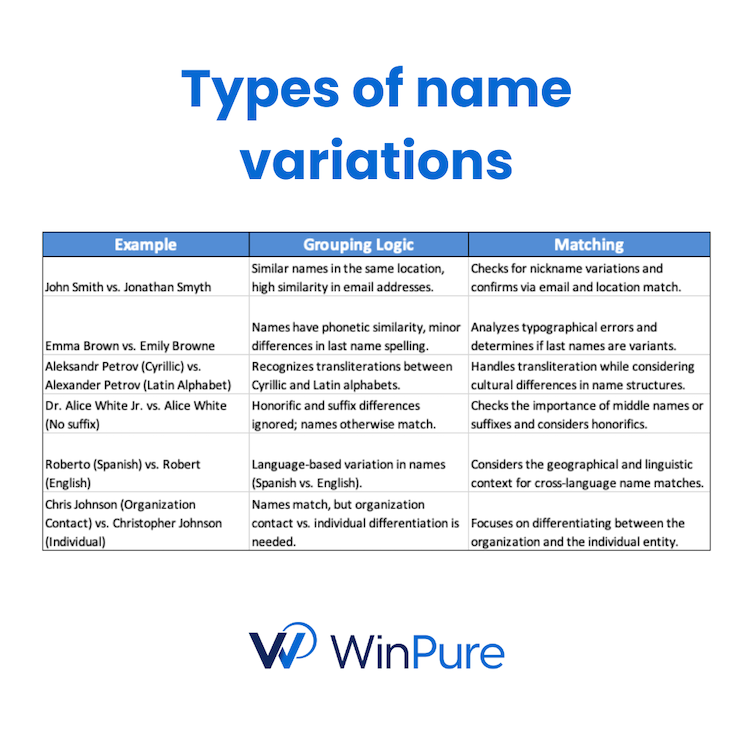
When dealing with such records, experts define the logic behind “matching” the data to derive the answer. Traditionally, the matching would be done manually using SQL and Python scripts to run matches. Technology has come a long way, however, and now AI-powered entity resolution engines can perform complex matches like the ones described above. They feature built-in principles that scan the data for these variations. These tools can identify complex duplicates without requiring any manual intervention from users.
Data Matching Is the Key to Entity Resolution
Entity resolution is achieved through a process called data matching.
Simply put, before you can answer whether Mary Smith and Mary Smyth are the same person, you first need to run a match on all available records. You’d need to either match for names or phone numbers to identify the number of duplicate records in the database. The match results should show you the different variations of the records that exist in the system. You’d then have to review the results and make a decision on which record is the most recent, reliable and updated. Once you’ve cleaned and matched records, you will then need to link or merge them into a single group. This means all the information that you need to unify all the information you have on Mary Smith into one column for easy referencing later.
The matching process is powered by algorithms that use string differences or phonetic variations to identify a match. For example, a phonetic-based data match algorithm will class Shawn and Sean as duplicates due to the phonetic similarity in pronunciation. Other algorithms will look at the similarities or differences between text characters. For example, Smith and Smyth are often phonetically the same but have a spelling difference of one character.
These algorithms, also known as fuzzy data match, are highly complex and require technical expertise. A company attempting to achieve entity resolution would have to hire trained developers or data scientists to build custom scripts for treating data. Due to the cost and complexities of such endeavors, many organizations are unable to resolve their data quality challenges, leading to compliance risks, ineffective marketing campaigns, disgruntled customers and poor operational efficiency.
Why Does Entity Resolution Matter?
Because business users are the true custodians of customer data. For example, between an IT person and an account manager, only the latter can confirm whether Mary Smith is the same person as Mary Smyth by taking into consideration factors like customer history, notes, exchanges and other information recorded in the CRM.
Furthermore, only business users understand the nuance of their contact data. For example, a marketing manager has more insight into why some records have country data while others don’t (for example, a local campaign may have only collected regional data). Therefore, when it comes to performing entity resolution, these business users must be aware of the context and disparities in data.
Additionally, because most organizations do not have data teams, it is up to business teams to perform entity resolution. The good news is, in the age of SaaS and no-code platforms, solutions have attempted to democratize the entity resolution process, making it accessible and easy for business users to take charge of their data in the absence of a dedicated IT resource. After all, marketing and sales teams can’t always ask IT to solve their CRM or customer data challenges!
Empowered with easy-to-use, no-code tools, both tech and business teams can now resolve their data challenges at scale. Tools and technologies are just one part of the process, however, companies need to prioritize data quality training and ensure their business users are kept in the loop when making decisions on data management processes..
It is high time businesses understood the basic building blocks of data. It’s not about putting together complex charts and visuals, but rather getting the numbers and text strings right to get a clear picture of their customers.