Are you building a startup and wondering if it’s the right time to hire a data scientist? Or are you a data scientist and wondering if you should apply for that job at an exciting new startup?
I get these questions a lot, so here’s what I’ve learned from working as a data scientist in a startup.
Is It Time for Your Startup to Hire a Data Scientist?
Understand the Stages of a Startup’s Lifecycle
Data scientists can help businesses with problem solving, innovation and scaling a business. However, when it comes to startups, it’s important to consider the company’s stage in the startup lifecycle. There are a lot of articles on the phases a startup goes through and most introduce a similar path.
The Stages of a Startup’s Lifecycle
- Problem-Solution Fit
- MVP
- Product-Market Fit
- Scale
- Growth
While not all startups move from one phase to another at the same pace, I want to point out the critical points you should consider at each phase. I also want to note that the definition of “data science” is not a universally agreed upon term. In this article, I use “data scientist” to mean those with a scientific mindset and the ability to build machine learning models. But as you’ll see below, data scientists in a startup will wear other hats, too, such as data analyst, data engineer and machine learning engineer.
It all depends on what stage the startup is in!
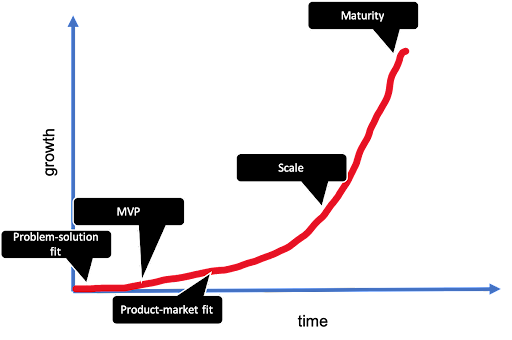
Stage 1. Problem-Solution Fit
For Businesses
Unless your business is to provide AI services, you don’t need data scientists at this phase. You’ve found a problem and you think you have a solution. You shouldn’t be thinking about data infrastructure and scalability at this point. You just need to do a lot of hands-on interviews and iterate until you get to the next phase.
But, if all your solutions involve AI services, you need a mature data scientist who’s aware of what can and cannot be done with AI solutions in production. This needs to be someone who is not into trending solutions and also doesn't jump into building AI services before they can see the big picture.
For Data Scientists
If you’re a data scientist and joining a startup in this phase, you’re one of only a few people in the company (possibly one of the co-founders!). At this point, you should expect no data science related tasks or maybe very general ones at this stage (such as data analysis with a bit of machine learning), unless the business solutions explicitly provide AI solutions. If that’s the case, you need to have a very good understanding of what can be done with AI without falling into all the hype. You’ll be one of the main decision makers in the startup so make sure you’re not jumping into building complex models just because you can. Also, you need to be aware of the risks; not all startups will be successful!
Stage 2. MVP
For Businesses
This is the stage to build the most viable product with the least amount of time and at the lowest cost. At this stage, you need an experienced data scientist who’s comfortable with working on loosely defined problems. They’ll help you accelerate some processes with your MVP, but don’t expect them to build something at this point that sets you apart from your competitors. This is just the MVP phase! You’ll need data scientists with a scientific mindset more than those who fall into the trap of MLOps. At this stage, MLOps will drastically slow down MVP iterations.
For Data Scientists
At this stage, deliverables are unclear, problems are loosely defined and data infrastructure is…non-existent. Expect to do a lot of analysis and work with small off-the-shelf ML models. Don’t expect the business to provide you with cloud solutions and a lot of data at this phase. You’ll have minimal data to train your models and minimal tools to work with. Don’t jump into implementing nuanced AI solutions at this point. Simple models and even heuristics can help the business learn and iterate quickly. Expect to do some parts of engineering and development tasks besides your data science work, but don’t fall into the trap of going deep into MLOps at this stage.
I once had to manually recommend items to users to understand what kind of recommendation engine we needed for our target users. We learned a lot of things we couldn’t possibly have learned by implementing a random recommendation engine.
Stage 3 . Product-Market Fit
For Businesses
This is a critical step for your data strategy. The business is finding the right market and will begin scaling soon. More importantly, if you have a two-sided marketplace, you need to scale to get indications of product-market fit. Therefore, you need data scientists to ensure you are collecting the right data in the right format. Look for data scientists who are comfortable with data engineering at this stage (if you don’t already have data engineers).
Suppose you’re building a search and recommendation engine and only collecting clicks, but not bounce signals. When you move to the scale phase and want help from AI services, you don’t have the correct data. More importantly, you need a data team with data engineers. At this point, you need to move away from passing excel sheets between teams, or you’ll be trapped when it comes to scaling!
Investment in data and data science is an example of J curve investment. It’ll be costly initially, and you won’t see meaningful ROI immediately. Product-market phase is the critical step for your data strategy.
For Data Scientists
Expect a lot of collaboration with the data team, engineering team and data analysts. You should be comfortable with engineering work and interacting with your less technically-minded colleagues to help make business decisions in the business by way of your data analysis. Don’t expect a big data and data science team at this point. You’re probably the only data scientist (or one of a few) in the company and still close to strategic business decisions. This means you’ll have a large field to discover on your own, as well as a lot of flexibility and responsibility for what comes next.
Stage 4. Scale
For Businesses
Now is the time to hire data scientists and expand the data team. Hopefully, you’ve started thinking and doing some of this work already. The data science team can help you scale many parts of the business. That said, you’ll still need a good understanding of what AI services to buy and what to build internally.
Data can be gold. So you need to understand what data is differentiating you from other competitors as you’re scaling. Don’t fall into the trap of hiring full-stack data scientists! No matter how good full-stack data scientists are, they will always manage some parts of the engineering work differently than engineers will. Hire people for their expertise at this phase rather than looking for those data science/engineering unicorns. In other words, hire data scientists with the niche skills that your business requires. Leave the engineering part to the engineering teams.
For Data Scientists
You can expect there to be a dedicated data engineering team to take care of ETL and data quality, as well as a dedicated team for data analysis. The company is probably also investing in proper data infrastructure; therefore, you can expect to work on problems that will help the business scale. This means there are real data science problems to solve! An important step in this phase is to make sure you are building feedback loops to make sure your machine learning models are improving as the business scales and more data comes down the pike.
Stage 5 . Growth
For Businesses
You have a mature data team with more data scientists, even possibly dedicated data science teams for various AI services you have in the business. You should have a culture of more data-informed decisions at this phase as you’re growing and expanding. You need to think of talent and retention at this phase, if you haven’t already. You may come up with specific problems that no other company has faced and therefore require extensive research to collaborate more with universities; you’re open to more innovation. At this stage, you need different types of data scientists: those with more science skills, data scientists with ML engineering expertise and more junior data scientists to help with analysis and feasibility phases.
For Data Scientists
You can expect a bigger data science team, which is possibly split into different teams. You probably won’t directly influence the strategic business decisions but there will be a lot of learning from other colleagues on various data teams. The company has matured in data infrastructure and you’ll have less influence over big changes to that infrastructure . However, you can expect to be able to go crazy and test state-of-the-art ML models to help with business growth.
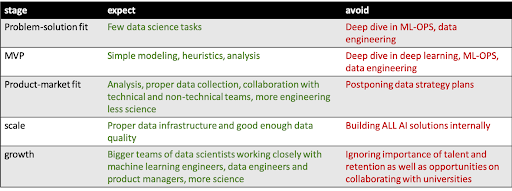
If you’re a data scientist and want to apply for a job at a startup, research where the startup is in their lifecycle. You will have very different experiences and responsibilities as the company grows.
As a startup, understand when to invest in data science and what type of data scientist is important for your business. It all depends on what stage you’re in.