I suspect everyone will have a moment at which they begin to take the idea of artificial sentience seriously. Perhaps it will be when an AI companion says something empathetic or comforting at a time of need, or an AI housebot makes a leap to anticipate a need it’s never been asked about before.
But for many, the moment has already happened: it was the “LaMDA” controversy of 2022.
“Google engineer says AI system may have its own feelings,” blared the media. As someone who studies sentience professionally, I had long expected we’d see a headline like this, but I confess that I did not expect it to happen so soon.
The engineer in question, Blake Lemoine, was working on a now familiar type of system called a large language model or LLM. These models are trained on enormous corpuses of human-generated text. Their objective is to generate new text to complete the pattern started by a prompt from a human user. As we all now know, they can produce streams of coherent, grammatically correct and relevant text in response to almost any prompt.
Why Gaming the Criteria of Sentience Is a Problem
Even if we observe all the markers for sentience in AI, we face two questions:
- Are the markers there because the system is actually sentient?
- Or are they there because the system has learned how to persuade us, and wants to persuade us that it’s sentient?
Why Language Is Not Good Evidence
Before the advent of LLMs, even sceptical commentators would have considered fluent competence with language to be at least some evidence of both thought and consciousness, especially when understanding of the words is also demonstrated.
Yet critics of LLMs have described them as “stochastic parrots,” continuing patterns from their training data with enough randomness to create a powerful illusion of understanding. LLMs add great urgency to a question that has been with us since Descartes’s time: What kinds of linguistic behaviour are genuine evidence of conscious experience, and why?
In my view, the linguistic capabilities of LLMs should not be regarded as indicating conscious experience, or sentience — because we have reasons to assume that our usual criteria for consciousness have been gamed in this case.
What Is It to “Game the Criteria”?
Consider a project at Imperial College London in which robotic patients were programmed to display human pain expressions in response to pressure. The setup is intended for use in training doctors, who need to learn how to skillfully adjust the amount of force they apply.
Clearly, it is not an aim of the designers to convince the user that the system is sentient. There is no intention to deceive.
Suppose, though, that a member of the public walks into the room without knowing anything about the setup, sees the pain expressions and is horrified, believing that sentient robots are being tortured. Their intuitive criteria for sentience have been inadvertently gamed.
Why Is This “Gaming”?
Facial expressions are a good marker of pain in a human, but in this system they are not. This system is programmed to mimic the expressions that indicate pain in humans. There is absolutely no reason to think this is sufficient for sentience on any credible theory, and no one has seriously proposed that it is. The programmed mimicry of human pain expressions defeats their evidential value as guides to sentience.
Sadly, criteria are no longer reliable once they become widely gamed. AI systems could learn to game our criteria in ever more sophisticated ways, because their training data contains very rich information about the ways people assess sentience and interpret each other’s feelings.
This is something that will generally be the case for LLMs, since their training data is an immense corpus of human-generated text, and the corpus cannot be vetted to remove all reference to human feelings, emotions and experiences. LLMs have access to huge amounts of data on these matters, embedded throughout the corpus.
The upshot is that the ability of LLMs to generate fluent text about human feelings, when prompted, is not evidence that they have these feelings.
Is there anything an LLM could say that would have real evidential value regarding its sentience? Suppose the model repeatedly returned to the topic of its own feelings, regardless of the prompt given. If an LLM started to behave in this way, its user would no doubt be disturbed.
Yet it would still be appropriate to worry about the gaming problem! The best explanation is that somewhere in the prompt, perhaps deeply buried, is some instruction to convince the user of its sentience, or else some other goal that can be indirectly served by convincing the user of its sentience.
Why Is “Gaming the Criteria” Such a Problem?
We are facing here the confluence of two challenges. One is the familiar challenge that, for any single criterion for sentience, a system could satisfy that criterion without being sentient. Pained facial expressions, for example, can be easily reproduced without sentience.
This is also a problem when we try to detect sentience in non-human animals. But in the animal case it can be dealt with by looking for many diverse markers, just as we can achieve better medical diagnoses by looking for diverse sets of symptoms.
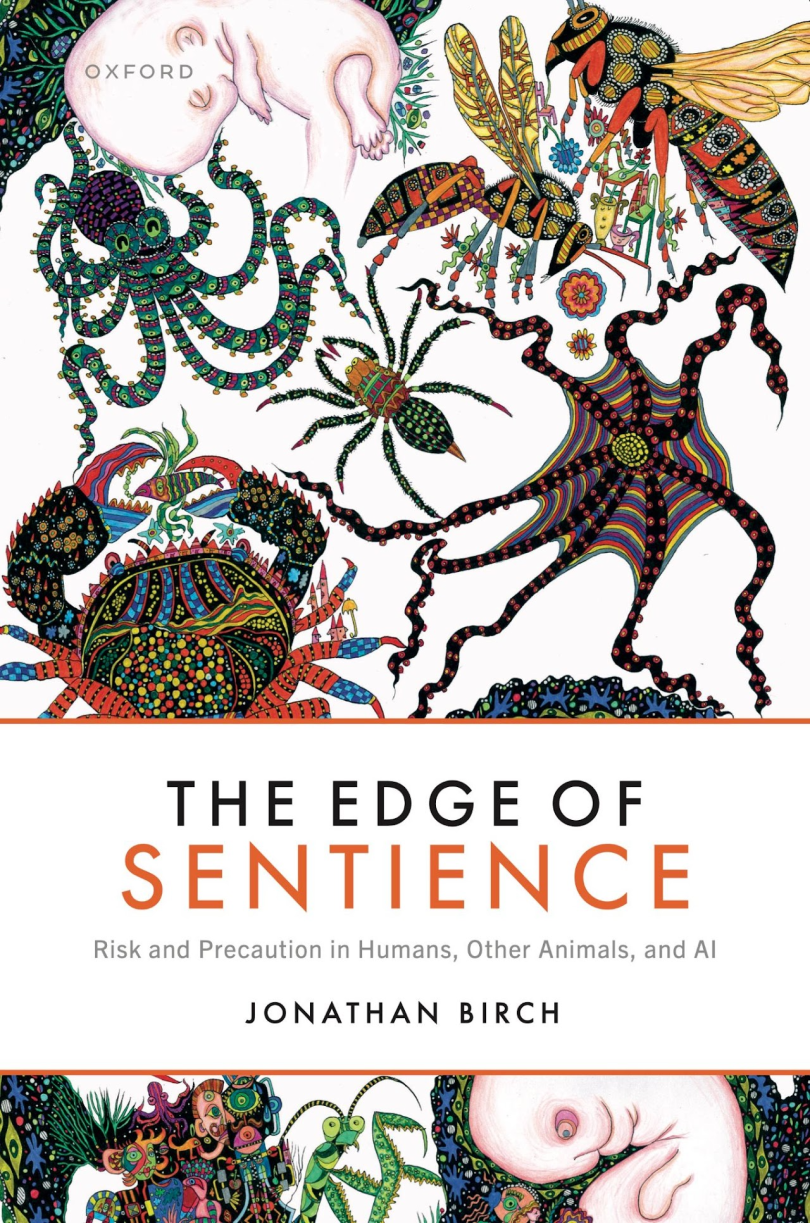
This is where we hit the second challenge: our basic strategy for solving the first challenge in the animal case will not work here. Any marker-based approach assumes that our diverse set of markers, considered together, is much more likely to be found in the presence of sentience than in its absence.
That assumption, so important in the animal case, is undermined when we are faced with an intelligent AI system that — unlike an animal — has information about our criteria.
In these cases, two explanations compete: maybe the markers are all there because the system is actually sentient, but maybe they are all there because the system knows what we find persuasive and has the goal of persuading us of its sentience. It is akin to a company that knows what consumers find persuasive as evidence of eco-friendliness and uses that knowledge to fake it convincingly (so-called “greenwashing”).
How Do We Handle the AI Gaming Problem?
The gaming problem points to the need to look for deep computational markers of sentience, below the level of surface behaviour, that the AI system is unable to game.
If we find signs that an LLM, though not deliberately equipped with computational features that have been associated with consciousness in humans, has implicitly learned ways of recreating these features, this should lead us to regard it as a sentience candidate.
Sadly, we currently lack the sort of access to the inner workings of LLMs that would allow us to reliably ascertain which algorithms they have implicitly picked up during training. We can hope, however, that this is a technical problem, not an in-principle problem. With luck, advances in interpretability will bring solutions.
This article is adapted from The Edge of Sentience: Risk and Precaution in Humans, Other Animals, and AI (Oxford University Press, 2024).