“I would say everyone has read at least once an algorithmically produced article,” said Robert Weissgraeber, CTO and managing director of AX Semantics.
Not everyone can tell, though. In many cases, readers don’t see a difference between human- and bot-authored copy, Weissgraeber told Built In. He would know. His company, AX Semantics, is one of several — including Narrative Science and Automated Insights — exploring natural language generation, or automated writing.
The technology can be used to generate product descriptions, quarterly earnings reports, fantasy football recaps and journalism. The Washington Post, for instance, has developed an AI-enabled bot, Heliograf, that helps generate election and sports coverage. Meanwhile, in Germany, where AX Semantics is based, the Stuttgarter Zeitung’s AI-augmented reporting on air pollution recently won a journalism award.
“We call it the Kasparov moment,” Weissgraeber said, comparing the win to the moment chess grandmaster Gary Kasparov lost a game to a supercomputer.
Human writers aren’t thrilled to be competing with algorithms. Their employment prospects were already fairly bleak. In 2019, almost 4,000 journalists — many of them writers — lost their jobs in a reckoning one writer termed “the media apocalypse.” This year, the coronavirus pandemic has prompted another industry-wide round of layoffs and furloughs.
“We’ve gotten death threats,” Weissgraeber said.
Does natural language generation spell the end of the already-besieged writing profession?
The Post newsroom doesn’t seem to think so. “We’re naturally wary about any technology that could replace human beings,” Fredrick Kunkle, a reporter for the Post and a co-chair of the paper’s union, told Wired of Heliograf. “But this technology seems to have taken over only some of the grunt work.”
Weissgraeber seconds this. AX Semantics’ technology, he said, is about “automating the boring part of the [writing] job,” he said.
“I always say it makes sure that you don’t have to do overtime.”
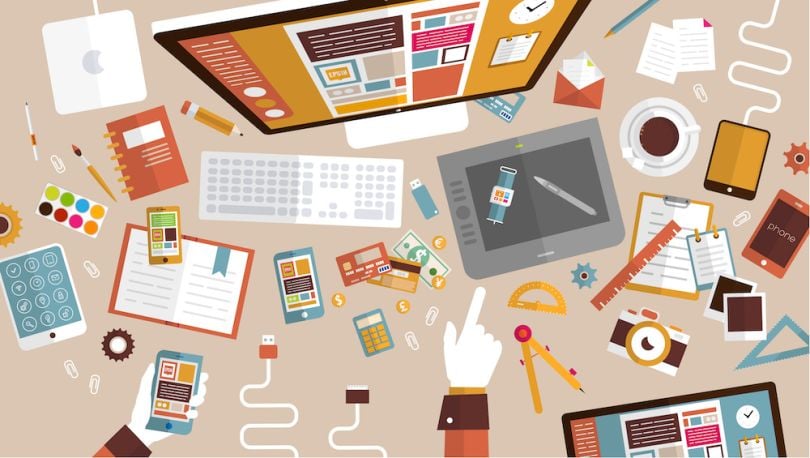
Writing at Scale
Natural language generation solves a core business problem with writing — it doesn’t scale very well. A writer can write one 1,000-word article in a week, no problem, but that writer can’t easily ramp up to 10,000 such articles per week when demand spikes. And in the internet age, the demand for content has spiked.
“Even if you’re a small e-commerce shop, you have 20,000 products and you have to be visible on Google and you have to conversion-optimize your text,” Weissgraeber said. Companies can’t reuse supplier copy, either — though readers don’t typically mind repetitive language, Weissgraeber said, Google’s search algorithms prioritize unique content.
So in the past few years, “the amount of content [needed] exploded.”
Natural language generation helps fill that need. Most current technology, Weissgraeber explained, operates in the “data to text” space, transforming structured data — like a cardigan’s size, style, material, brand and price — into a piece of prose. Here’s what that can look like:
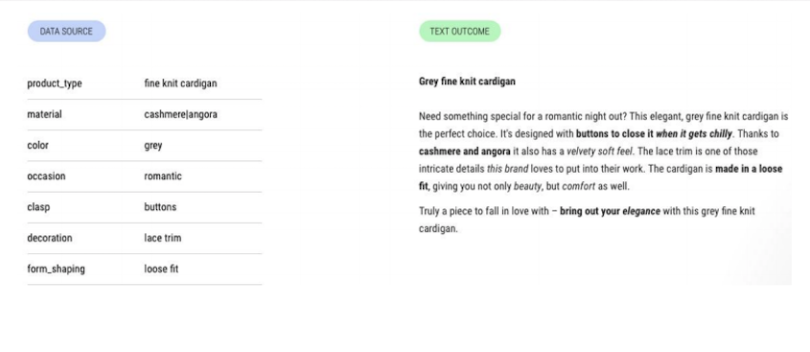
A human could pen that “text outcome” too, obviously, but they couldn’t easily scale it to 2,000 almost-but-not-quite identical cardigans, or translate each of those descriptions into 20 languages. Weissgraeber estimates a project like that would take a team of at least 20 writers, translators and editors — and they’d be bored out of their minds. Natural language generation can automate most of that process, though. (AX Semantics’ software can also translate text into 110 languages.)
Conversion optimization presents similar issues. AX Semantics’ clients often ask if they should use formal or informal language in their online stores, Weissgraeber said. Testing to figure that out can require new, tonally tweaked versions of thousands of product descriptions. That would take humans ages to produce. AX Semantics’ natural-language-generation software, though, can shift text from formal to informal at the push of a button.
Needless to say, this wasn’t always possible.
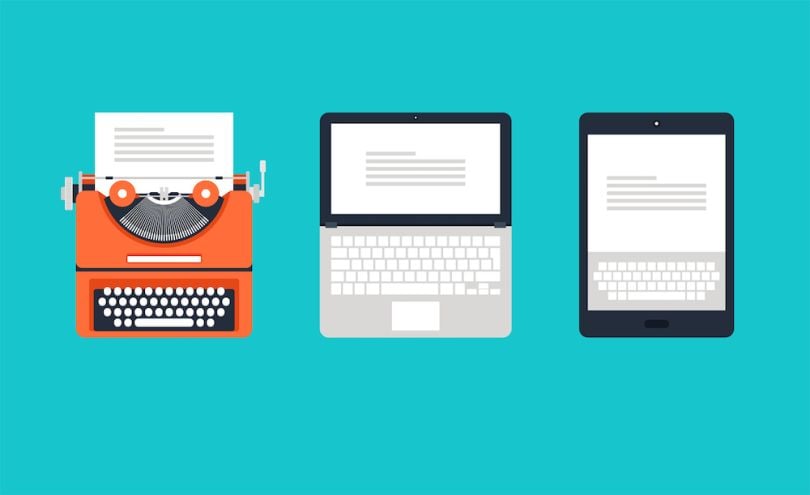
From Templates to AI-Enabled ‘Micro-Decisions’
Early natural language processing looked more like Mad Libs than revolutionary technology. The earliest attempts at it were template-based systems for writing local weather forecasts. These systems were automated, but in a fairly rote way — they essentially plugged new numbers into old prose, Weissgraeber said.
Some forecast-generating attempts date back to the 1980s, but even by the aughts, natural language generation hadn’t evolved that much. When StatSheet debuted in 2007, it automatically published real-time data on college basketball games and players. It was still template-based natural language generation — it had just migrated onto the web.
These systems started getting smarter about five years ago, though, according to Weissgraeber. AI advanced to a point where it could learn languages without extensive manual configuration. Instead, algorithms could simply ingest reading materials in a given language, and “learn” that language autonomously from the unstructured data. This made natural language generation less effortful. AI-enabled natural language generation software could translate quickly, check its own grammar, surface synonyms to ensure a text’s uniqueness and control its tone.
This was a powerful upgrade, but natural language processing still isn’t as powerful as a human writer. To return to the example of a cardigan: It’s obvious to a human what a cardigan is, but AI has no idea what the word signifies, what place a “cardigan” occupies in our culture, or why a cardigan has buttons.
“You have to teach the system what features mean,” Weissgraeber said. “So if you’re writing about a cardigan and it has buttons, you tell it what they’re for — to close the cardigan, so you can stay warm when it’s chilly.”
This type of knowledge, or “domain expertise,” has to be added into natural language generation software manually — but it only has to be added once, in the form of an if-then statement. (For instance: IF “buttons,” THEN “You can button it up on chilly nights.”) Once the user creates and prioritizes enough if-then statements, the software can make appropriate “micro-decisions,” Weissgraeber said, and pen thousands of cardigan descriptions.
In other words, a person still has to effectively “write” the first cardigan description — but natural language generation can turn that into thousands of descriptions.
The published descriptions can remain linked to a back-end database too — the “data” piece of “data-to-text” — which means that the text updates whenever the database does. AX Semantics calls this feature “live editing.” This not only means that fixing back-end errors automatically fixes front-end copy errors, but also that superlatives update constantly. So if an e-commerce shop touts one cardigan as its cheapest and then starts stocking a new, even cheaper cardigan, the “cheapest” tag moves to the new offering automatically.
Human copywriters just can’t offer that level of long-term accuracy. Nor should they be asked to! But they can offer one thing AI still can’t: creativity.
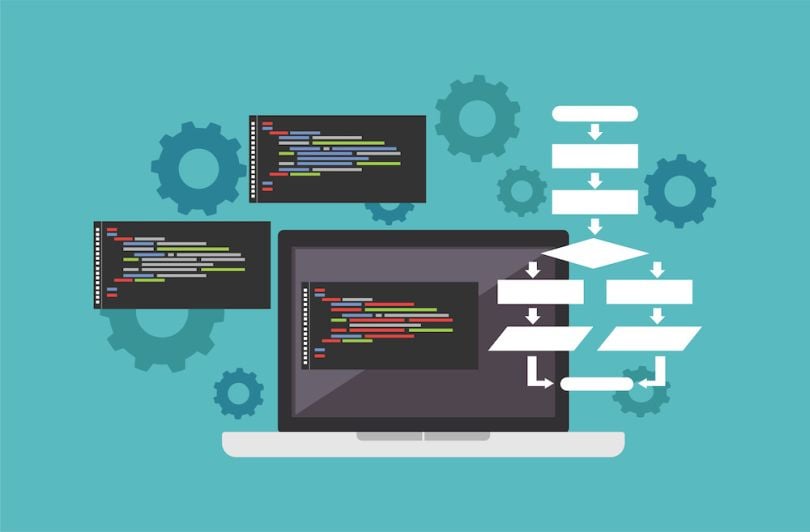
The Future of Algorithmic (and Analog) Writing
“The current state of the art [of natural language generation] is that you either have creativity or control,” Weissgraeber explained.
AX Semantics focuses primarily on control — which means a manual set-up process. But some other tools in the field focus more on creativity. Talk to Transformer, for instance, can generate a chunk of text based on a brief prompt, but the AI doesn’t actually know the meaning of what it’s saying. It’s just putting things together words that it has seen together before.
A human can tell in a heartbeat. When we prompted Talk to Transformer with “You’ll love this cardigan,” here’s what it generated:
You’ll love this cardigan! When I tried to find this softest cardigan out there I was disappointed at first due to not having this pattern. I couldn’t find it anywhere on the web. In fact I came upon the patterns for the zip up sweatshirt and the button up jeans, but I had no idea there was a pattern for this cardigan. It wasn’t until I picked up this recipe that I realized there is a basic knit/purl pattern in this baby blanket. As I am not a knitter I found it much easier to modify the basic pattern and added different sizes to the blanket to make it baby sized, men’s size and toddler sized. I worked on the top stitch pattern to make it easier to follow for beginner knitter.
This is very creative, but not particularly functional. Weissgraeber doesn’t see this type of open-source AI striking out on its own. Instead, he foresees a rise of “hybrid content,” resulting from a collaboration between humans and AI. AX Semantics’ software enables this; so does the Washington Post’s Heliograf.
There’s plenty of room for growth, though. Readers may have all seen algorithmically penned text, but Weissgraeber is careful to distinguish this from widespread adoption of natural language generation software. He estimates that about 1,000 companies use NLG products — and though they use them “broadly,” that’s still “basically nothing” in terms of a user base. The field’s nearest-term challenge, he said, is attracting new clients, who will introduce new use cases and inspire new features.
Weissgraeber also sees AI and automation growing more powerful. Though algorithms can’t conduct their own research anytime soon, in a decade, he predicts they’ll be able to generate research papers from outlines, to the chagrin of teachers everywhere.
It feels worth noting, in closing, that I am human and wrote this article without AI assistance.