If you thought AI was mostly being used by top-level executives and ambitious engineering interns, think again.
According to a survey conducted by the Federal Reserve Bank of St. Louis, 40 percent of Americans between the ages of 18 and 64 use AI, specifically generative AI, to some degree. While the study shows that this usage occurs both within and outside of the home, the majority of users claim to rely on Gen AI the most when they’re at work.
So how are employees using AI in the workplace? According to technologists at companies across the country, AI’s impact takes many forms.
At Capital One, for instance, AI and ML are being used to identify fraud. Meanwhile, at Roblox, the technology plays a key role in delivering a more enhanced user experience.
Regardless of the approach taken when leveraging AI, the end result for these two companies and many others is the same: make a greater impact on the tech industry – and the world at large. Read on to learn how tech teams at 15 companies are using AI and ML to enhance the product development process and how each of these organizations keeps up with advancements in this space.
Capital One offers financial services for both consumers and businesses, ranging from credit building to automotive financing.
How is your team integrating AI and ML into the product development process, and what specific improvements have you seen as a result?
My team is responsible for investing in emerging research in the AI and ML space and applying it to the financial context. We do this by working closely with our tech partners and teams across Capital One to identify common modeling challenges within the business. Additionally, we accomplish this through applied experimentation to identify the best AI and ML techniques to solve a particular business challenge and scale the impact so it can be used across the company.
Our team has helped introduce unsupervised and graph ML techniques to support our customers and the business in various areas, such as identifying fraud, customer service needs and providing personalized app experiences. This work has helped reduce the time data scientists spend on customized feature engineering, allowing them to move quickly and efficiently deliver value to customers sooner.
What strategies are you employing to ensure that your systems and processes keep up with the rapid advancements in AI and ML?
My team works closely with our partners to deliver on Capital One’s AI and ML strategy, which includes thinking about responsible applications of AI. Across all of our work, we’re guided by a mission to build and deploy AI and ML in a well-managed way that puts people first. This includes working cross-functionally across the business to follow best practices, such as extensive testing and implementing human-centered guardrails before introducing AI systems or models into any customer or business setting.
We also ensure that we only use AI and ML to solve a problem when it’s the right way to solve the problem. We see that, in some situations, business logic is sufficient enough to solve the problem at hand. We focus our efforts toward making an impact on our business rather than trying to use something flashy just for the sake of saying we use AI.
“Across all of our work, we’re guided by a mission to build and deploy AI and ML in a well-managed way that puts people first.”
Can you share some examples of how AI and ML has directly contributed to enhancing your product line or accelerating time to market?
By partnering with teams focused on fraud, we’ve been able to use ML techniques to make big breakthroughs in our ability to mitigate fraud and help protect our customers. Using AI and ML, we’re better able to adapt to emerging changes in behavior patterns as fraudsters constantly change their techniques, greatly improving our detection of anomalous behavior. We’re continuing to experiment with new AI and ML capabilities to stay at the leading edge of this space and continue to give customers the best possible experience.
Roblox’s platform enables people and organizations to create and share immersive gaming experiences.
How is your team integrating AI and ML into the product development process, and what specific improvements have you seen as a result?
In 2021, we began building our AI platform. At that time, the lack of a unified Roblox AI platform led different teams to build individual platforms and use a variety of frameworks. Each team had to develop its own optimizations and tackle scaling challenges independently.
As a result of building our unified platform, we saw tremendous acceleration in our AI products. In early 2023, we supported fewer than 50 ML inference pipelines. Today, our infrastructure supports approximately 250 of these pipelines.
What strategies are you employing to ensure that your systems and processes keep up with the rapid advancements in AI and ML?
We’re committed to being strong partners to the open-source AI community. We leverage open-source projects, leveraging Kubeflow in our ML pipelines, batch inference workloads and feature store. We also contribute to vLLM, an open-source library for inference and serving for large models. We recently announced our first open-source model — our ML voice safety classifier — and are currently working on open-sourcing our 3D foundational model. Being involved in the open-source community helps us keep up with ever-evolving advances in AI.
“Being involved in the open-source community helps us keep up with ever-evolving advances in AI.”
Can you share some examples of how AI and ML has directly contributed to enhancing your product line or accelerating time to market?
Interactions on Roblox are powered by AI. For example, when a user logs into Roblox and looks at which experience to join, AI is at work through our recommendation and search systems. When that person chooses an experience and hits the play button, our match-making algorithm identifies the best server to join. Once a user is in a server, automatic chat translation helps people who speak different languages communicate and connect with each other.
Today, millions of creators have access to our Gen AI tools. Assistant is a conversational AI tool that helps creators learn, code and build more efficiently by automating tedious, repetitive tasks. With our Texture and Material Generator tools, creators can quickly change and iterate on the look and style of objects. And we’re now entering the era of 4D Gen AI with the recent launch of Avatar Auto Setup, which simplifies avatar creation, saving creators hours or even days of work by automating all the stages in the process.
SailPoint’s identity security platform helps organizations mitigate cyber risks.
How is your team integrating AI and ML into the product development process, and what specific improvements have you seen as a result?
At SailPoint, we think about incorporating AI in a few distinct capacities. The first is internal productivity. For example, many of our engineers use AI-powered coding tools. We’ve observed improvements in the form of reduced cycle times to complete tasks and found that developers who use these tools feel both more productive and more fulfilled by their work. My teams have also used AI to summarize meeting notes, generate documentation for code, create synthetic data for experiments or automated testing and build internal knowledge bases that enable faster and more targeted retrieval of technical information. We’re continuously exploring ways to apply AI to make ourselves more efficient and better at what we do.
The second is incorporating AI directly into our products to help improve the overall experience and security posture of our users. SailPoint knows from experience that identity security is difficult. Organizations are dynamic. Organizations evolve, and each one’s security and compliance efforts need to evolve as well. AI provides a mechanism to help extract important insights at scale and continuously adapt to the changing security needs of our users.
What strategies are you employing to ensure that your system and processes keep up with the rapid advancements in AI and ML?
The first priority was to cultivate a robust data infrastructure. AI relies on high-quality and accessible data. SailPoint has developed and matured that infrastructure over time and continues to invest in it. Having data in a central location with mechanisms for governance and efficient access enables faster experimentation with new tech.
We also invest in our own infrastructure, tools and environment to build and deploy ML models. This includes components, such as our model registry, which is used for version control of our models and to enable collaboration between our engineers and our feature store, which allows us to track and reuse features across different models. Together, these types of components allow us to quickly integrate new advancements.
There’s an organizational component as well. We employ a hybrid structure that encourages engineers to build expertise in specific domains and adapt to their local needs while also establishing centralized AI resources and standards. We also provide avenues for collaboration so that our engineers can integrate new technologies relevant to their domain first and then bring that knowledge back to the broader group.
“We employ a hybrid structure that encourages engineers to build expertise in specific domains and adapt to their local needs while also establishing centralized AI resources and standards.”
Can you share some examples of how AI and ML has directly contributed to enhancing your product line or accelerating time to market?
A key metric at SailPoint is time-to-value for our users. We recognize that identity security can feel like a heavy lift, and we want to make that user journey as seamless as possible. That starts on day one with an efficient setup. Many businesses have hundreds or even thousands of applications they want to integrate with our identity security solution. This involves a significant amount of manual effort. We recently introduced an AI-powered application onboarding capability, which automates the discovery of applications and provides configuration recommendations based on usage patterns observed over time. This helps our users get up and running faster.
We’ve also incorporated AI capabilities into our product line to help users implement effective identity security strategies at scale. For example, Role Discovery analyzes access patterns within an organization and groups access into roles that can be more easily assigned and managed. Identity Outliers enables administrators to more quickly discover and remediate risky access. These innovations help empower organizations to enhance their security posture and focus more on their core business objectives.
Upstart’s AI-powered lending marketplace connects consumers with banks and credit unions, gauging credit worthiness on education and employment rather than solely on credit scores and income.
How is your team integrating AI and ML into the product development process, and what specific improvements have you seen as a result?
At Upstart, ML and AI models are the heart of our product. In fact, you could argue that these models are our product. For example, our underwriting models drive the loan offers we provide on our platform and are designed to evaluate the credit risk of any given applicant. That is, they answer the question, “What is the likelihood this applicant pays back the loan?” For our product to succeed, we must do this with a high degree of accuracy. To achieve this, our underwriting models utilize state-of-the-art ML techniques trained using a vast amount of payment data and alternative credit variables that many lenders overlook. Beyond underwriting, we use ML models to optimize marketing spend and detect financial fraud.
A major aspect of our product development is working on improving these models. This can take the form of integrating new data sources or developing new modeling techniques. We continuously update our models to keep up with economic trends and improve performance. The process involves ML researchers, ML engineers, risk reviewers and, of course, product managers. This results in higher approval rates and a seamless experience, with funds often reaching customers in a few days.
What strategies are you employing to ensure that your systems and processes keep up with the rapid advancements in AI and ML?
First, we hire bright and highly motivated people. One of our core values is “Be smart, but know you might be wrong.” Our people are encouraged to try new things, such as new modeling techniques, implementing new training infrastructures and thinking outside of the box about how the product works. This encourages an environment of continual learning that allows us to keep our team’s knowledge at the forefront of AI and ML.
Next, we’re provided with access to the best computational resources. Modern ML and AI can be constrained by the amount of compute required to implement it, both in training the models and serving them in production. This requires investing in compute resources to allow for fundamental research that strives to continuously improve our models. Finally, we have a dedicated set of engineers and risk professionals who support our AI and ML researchers in building robust frameworks for training, maintaining and deploying models in a cost-effective manner.
"Our people are encouraged to try new things, such as new modeling techniques, implementing new training infrastructures and thinking outside of the box about how the product works."
Can you share some examples of how AI and ML has directly contributed to enhancing your product line or accelerating time to market?
Upstart takes a unique approach to underwriting where we rely primarily on sophisticated ML models to make decisions on how to price loans. Alternative approaches include using a human to manually review credit applications and apply predefined rules or applying a more traditional statistical model to assess credit risk. The first approach is clearly costly since it directly involves a trained person to review each loan application. It’s simply not scalable. The second approach, while closer to ours, doesn’t achieve the accuracy required to meaningfully expand access to credit to underserved consumers. By utilizing better, more accurate models, we can approve more consumers at lower rates all while maintaining loan performance.
iManage’s platform enables organizations to uncover and activate knowledge that exists across various forms of content and communications in order to work more effectively and securely.
How is your team integrating AI and ML into the product development process, and what specific improvements have you seen as a result?
Businesses understand that data is critical to success and the future, but the vast amount of data flowing through these organizations every day can often overwhelm rather than empower knowledge workers. At iManage, we focus on integrating AI into our products to directly address this challenge. Our AI solutions are designed to cut through the noise and activate the collective knowledge stored within the world’s top knowledge work organizations, enabling knowledge workers to access insights in seconds, whether it’s knowledge assets, such as precedents and guides, or the experts who created those assets. By embedding AI across our product suite, we ensure that this crucial knowledge is always at the user’s fingertips, allowing them to focus less on the mundane and more on their clients.
What strategies are you employing to ensure that your systems and processes keep up with the rapid advancements in AI and ML?
While AI is transforming the legal tech landscape, the core principles of understanding user needs and solving real-world problems remain constant. Our approach begins with a strong emphasis on information architecture before AI. This means we focus on building a solid foundation by carefully structuring and connecting data in a way that aligns with user needs. By doing this, we enable our AI-enabled products to deliver precise, actionable insights and ensure the right knowledge reaches the right person at the right time.
Equally important is the continuous evaluation of the state-of-the-art. We constantly test the latest developments in AI to fully understand both its strengths and its limitations. This helps us design systems that take advantage of AI’s capabilities while addressing its weaknesses. By pushing the boundaries of what’s possible and refining our understanding of the technology, we ensure that we’re always implementing AI in a way that adds real value to our users. This combination of a strong data foundation and ongoing evaluation ensures that our systems are not only capable of keeping up with AI’s rapid evolution, but also provide practical, everyday solutions.
“We constantly test the latest developments in AI to fully understand both its strengths and its limitations.”
Can you share some examples of how AI and ML has directly contributed to enhancing your product line or accelerating time to market?
A prime example of how AI has transformed our product line is document enrichment. We use AI to automatically enrich every document uploaded to the DMS with crucial attributes, such as document category, parties, jurisdiction, key dates and more. This automated process not only makes documents far more searchable and provides users with immediate, context-aware results, but it also relieves our customers from the time-consuming task of manual data entry.
By automatically structuring and connecting data, we ensure quality and enable knowledge to flow seamlessly through organizations. This allows our customers to unlock their collective knowledge, making it accessible across teams and departments, which enhances collaboration and drives smarter decision-making. In essence, we’re empowering organizations to make the most of their data, ensuring that it’s not just securely stored, but also actively used to create value.
Opendoor’s platform enables consumers to buy and sell properties and view insights on the real estate market.
How is your team integrating AI and ML into the product development process, and what specific improvements have you seen as a result?
We’re integrating AI across both engineering enablement and product development. On the engineering side, we’ve invested in Gen AI-based code generation tools to accelerate developer productivity. Additionally, we’ve implemented AI-driven enterprise search capabilities using large LLMs to quickly summarize, analyze, discover information and derive insights and actions. This not only speeds up decision-making, but also enhances the quality of our design documentation by providing a deeper understanding of organizational touchpoints.
On the product side, we’re exploring various AI-powered enhancements to improve the customer experience and engagement. We’re investigating the integration of a chatbot functionality to provide more responsive customer service, and we’re utilizing vision and text Gen AI capabilities to increase transparency and trust with our users. Furthermore, we’re developing co-pilot features for our operations teams, enabling team members to more effectively explain our product offerings to potential customers, ultimately improving conversion rates and fostering trust.
What strategies are you employing to ensure that your systems and processes keep up with the rapid advancements in AI and ML?
To stay ahead of the rapid advancements in AI and ML, we’ve adopted a multi-pronged strategy. Each department evaluates AI opportunities relevant to that unit’s specific functions, which go through leadership reviews and are aligned with overall organizational strategy. Each of these tactics are ruthlessly prioritized and few are short-listed to experiment on. Organizationally, we host regular “brown bag sessions” to keep our teams up to date on the latest technologies. We’re also collaborating with vendors and strategic partners to solicit and enhance AI capabilities across both product development and internal enablement capabilities.
We prioritize thoughtful implementation by leveraging our governance, risk and compliance function to assess potential risks, including data security and ethical considerations. Additionally, our experimentation platform plays a key role in identifying and scaling the most impactful AI initiatives, ensuring that we double down on innovations with the greatest potential.
“We prioritize thoughtful implementation by leveraging our governance, risk and compliance function to assess potential risks, including data security and ethical considerations.”
Can you share some examples of how AI and ML has directly contributed to enhancing your product line or accelerating time to market?
The Opendoor Valuation Model is our core capability that powers our home pricing features and enables us to provide millions of cash offers to homeowners across the country. We use techniques, such as LLMs, adversarial networks, quadratic programming, convex optimization, time-series forecasting, Bayesian networks, computer vision, speech recognition and deep learning. These techniques help in extracting useful information from raw data, optimizing processes and improving the customer experience.
A second example is our usage of GitHub Copilot. This helps our developers by generating code snippets, functions and entire modules based on comments and code context, which reduces the need to write repetitive code. Copilot also aids in debugging and provides support for unit tests, making it easier to understand and maintain the code. Additionally, it offers good documentation for the generated code, enhancing code quality and maintainability. By automating repetitive tasks, developers can focus on more complex and creative aspects of their work, increasing overall productivity.
Empower helps consumers access cash and build their credit history, using bank data to see a fuller financial picture than through a credit score alone.
How is your team integrating AI and ML into the product development process, and what specific improvements have you seen as a result?
As a credit company, we’re incorporating AI and ML primarily from a data science angle. We rely on a number of models to help us translate vast amounts of data into innovative financial products and actionable feedback. These models impact how we design, build features and match customers with the right product. We’re also looking to these models for insights post-launch and to help inform our roadmap going forward.
I think one of the best improvements has been our speed. For example, we created a brand new product: Thrive, Empower’s line of credit, without time lost to testing before we built a model. Instead, we used novel techniques to create a proxy for a longer-term risk model. Traditional methods would require at least six months of lead time, but we were able to get this into market immediately. While we remained thoughtful and controlled with the rollout, our ability to iterate quickly enabled us to bring the product to general availability much faster.
What strategies are you employing to ensure that your systems and processes keep up with the rapid advancements in AI and ML?
There are no real shortcuts to creating a resilient, robust credit company, but there are certainly optimizations in getting there. That’s how I think about our use of these tools. Models can be incredible aids for us, but there’s a time to move fast and a time to move slow. We have an open dialogue across our credit and data science teams to discuss new, emerging resources, but we’re also carefully validating any additions. The newest, flashiest tech doesn’t always make sense, and in certain cases, it might take up to 12 months to realize a model has big flaws. We’ll always test and optimize our existing infrastructure, but our biggest focus is on the quality of data that feeds into our modeling.
“We’ll always test and optimize our existing infrastructure, but our biggest focus is on the quality of data that feeds into our modeling.”
Can you share some examples of how AI and ML has directly contributed to enhancing your product line or accelerating time to market?
I’d argue that our cash advance product models are among the strongest in the industry. We can understand risk for a single loan in two to four weeks. For traditional credit, this can take closer to two to four years. Of course, our loans are much shorter-term, but AI- and ML-informed modeling helps us move faster and with more accuracy. We can underwrite customers more thoughtfully and can serve them the right product at the right time.
We’re also able to quickly create and rebuild our models in a kind of continuous deployment cycle. We can refresh a model with more recent data and have a readout in weeks rather than months or years, as is the case at larger companies. I think having this rapid iteration loop is a really cool and powerful resource and definitely gives us an edge to learn fast and move quickly.
Work & Co is a design and technology company that partners with companies, including IKEA, Apple, PGA TOUR, Gatorade, Google and more, to launch digital products that transform businesses.
How is your team integrating AI and ML into the product development process, and what specific improvements have you seen as a result?
We’ve adopted a human-centered approach to AI integration that enhances, rather than replaces, our team’s capabilities. We developed a custom internal chatbot powered by OpenAI’s LLMs, giving our team a secure environment to leverage AI while protecting sensitive client information and democratizing AI access across all disciplines.
Our developers have embraced tools such as GitHub Copilot and AI-enabled IDEs like Cursor, all of which have accelerated common development tasks, such as boilerplate code generation, API integration and test writing, empowering our team to focus on more strategic and exciting aspects of product development.
We’ve enabled AI features on Notion, which we use as an internal knowledge base and collaborative documentation platform for client deliverables. Integration of an LLM into the editing workflow lets us prioritize the substance of our work rather than focus on wordsmithing and formatting.
Hands-on experience with a wide spectrum of AI tools, models and platforms has enhanced our ability to conceptualize and build similar AI-powered solutions for our clients — from efficient internal tools that optimize operations to highly personalized, intelligent B2C products.
What strategies are you employing to ensure that your systems and processes keep up with the rapid advancements in AI and ML?
Our approach to staying current with AI advancements is multi-faceted. For client work, we typically adopt a composable architecture strategy, designing systems with modular components that can be updated or replaced as the underlying technology continues to evolve. This flexibility has proven crucial in areas like image generation, where we can transition between different models — this includes the recent shift away from Stable Diffusion and toward Flux — without time-consuming refactoring work.
We learn by shipping products. We engage directly with AI technologies and test, iterate and refine in real-world scenarios. This helps us gain experience and deepen our understanding of new technologies as they emerge and often lets us offer our clients a distinct first-mover advantage.
Knowledge-sharing is critical to keep up with the fast pace of change. Our monthly “What’s New in AI” sessions foster cross-company dialogues, so we can share our collective expertise and stay ahead of industry developments. We invest in our team’s growth through continuous learning opportunities, helping us maintain a forward-looking perspective on AI advancements and each one’s practical application in our work.
"We engage directly with AI technologies and test, iterate and refine in real-world scenarios."
Can you share some examples of how AI and ML has directly contributed to enhancing your product line or accelerating time to market?
Our recent collaboration with Gatorade and Adobe — a path-breaking Gen AI-powered customization experience on the Gatorade iD membership platform — is a prime example of AI’s impact on the products we build. By integrating Adobe Firefly, we’ve transformed the traditional Gatorade Squeeze Bottle into a canvas for personal expression. Athletes can create unique bottles by simply describing their vision, whether inspired by their sport, personal interests or artistic preference, ensuring each bottle is as individual as the athlete who carries it.
With Adobe Firefly Services APIs, we’ve enabled the generation of on-brand designs that maintain Gatorade’s iconic aesthetic and unlock unprecedented creative freedom. The customization experience is seamlessly integrated into Gatorade.com, where members can earn points to generate bottle designs or draw inspiration from preset options, making personalization both engaging and accessible.
The overwhelmingly positive response and packed booth lines at this year’s Adobe MAX conference validate our approach to using AI to create deeply personalized experiences and get granular in tailoring each product to match an individual user’s taste.
Trumid’s credit trading network is designed to provide an optimized trading experience, offering real-time analytics, protocol flexibility and more.
How is your team integrating AI and ML into the product development process, and what specific improvements have you seen as a result?
As a leading provider of electronic trading solutions for the capital markets, our initial efforts to deploy AI and ML focused on embedding intelligence into existing features of our trading platform. From an engineering perspective, this approach was initially challenging, as many of our systems weren’t designed with ML use cases in mind. However, more recently, we have shifted to treating AI and ML as first-class considerations right from the ideation phase of new products. This change has allowed us to focus our resources on building more effective models and creating exciting opportunities to better serve our clients and advance our products and solutions.
What strategies are you employing to ensure that your systems and processes keep up with the rapid advancements in AI and ML?
We believe that innovation comes from all areas of the company, not just those directly working on AI and ML. To foster this, we’ve made development environments available to all engineers at Trumid, where they can access the latest Gen AI models and prototype their ideas. This inclusive approach empowers everyone, from front-end engineers and DevOps team members to data engineers and ML researchers, to stay current with model advancements and quickly test their use cases without significant technical barriers.
“We believe that innovation comes from all areas of the company, not just those directly working on AI and ML.”
Can you share some examples of how AI and ML has directly contributed to enhancing your product line or accelerating time to market?
One significant enhancement we’ve made is upgrading our notification system, which alerts users to time-sensitive trading opportunities. We’ve integrated an ML model that scores each opportunity as it’s identified, enabling us to filter notifications so users only receive those that are highly relevant based on their trading objectives. By increasing the success rate of notifications that users engage with, we’re able to deliver more valuable, targeted insights to our clients, ultimately enhancing their platform experience and potential trading outcomes.
Jabra Hearing offers hearing aid solutions and a mobile app that allows customers to manage the product’s settings.
How is your team integrating AI and ML into the product development process, and what specific improvements have you seen as a result?
It’s an exciting time for our product development teams in the world of AI, or more specifically, Gen AI. There now exists numerous Gen AI solutions available to us to help accelerate our development process and, more importantly, speed up and reduce manual effort with some of the more tedious aspects of our jobs. Our team is super excited about leveraging Gen AI solutions, and I’m so happy to be partnering with them to reap the benefits. Our engineering teams now have the ability to learn and gain expertise in new technologies much faster and with less effort than ever before.
We were recently able to automate several time-consuming processes by asking for some help with a scripting language that our team wasn’t already familiar with. Our team was able to quickly generate and get an understanding of the required script using Gen AI much faster than learning it from scratch, and our entire department is now reaping the benefits of having the automation in place. Another example is using Gen AI to help us generate test cases and testing frameworks using our own requirements as inputs rather than manually creating test frameworks from scratch.
What strategies are you employing to ensure that your systems and processes keep up with the rapid advancements in AI and ML?
The Gen AI solution space is crowded. Our strategy for leveraging AI and ML includes an analysis of the market and selecting proposed solutions that best integrate with our existing product development processes and tools. We look at how a proposed Gen AI solution meets our requirements for data privacy and cybersecurity. As a healthcare company, data security, privacy and compliance are critical requirements for us, and not all AI solutions are going to be compatible with our needs. We will then develop a proof-of-concept to validate our proposed solution.
Solutions such as built-in AI features of existing tools and platforms and those with straight-forward integrations with our existing data platforms will undoubtedly get a higher priority than others. AI solutions are only as good as the data they have access to; ease of integration with existing data systems is a key component of our strategy. Leveraging LLMs on top of our own data is extremely powerful. Fostering a culture of continuous improvement is key to our strategy to identify AI opportunities; weighing the benefits, fit with existing systems and requirements and cost are key to our decision-making.
“AI solutions are only as good as the data they have access to; ease of integration with existing data systems is a key component of our strategy.”
Can you share some examples of how AI and ML has directly contributed to enhancing your product line or accelerating time to market?
The highest priority use of AI and ML for us in product improvement is to leverage these technologies in combination with the breadth and depth of data that we’ve collected over the years, such as hearing aid usage, diagnostic and performance data, fitting information and customer experience information. Utilizing Gen AI and ML on top of our wealth of hearing data allows us to improve outcomes for our customers. It would enable our users to hear the world around them more effectively by optimizing their experience, proactively suggesting changes to programming in our Jabra Enhance Select mobile app, fitting and suggesting maintenance based on a deep understanding of the data and utilizing the predictive capabilities of ML.
By recognizing certain patterns of performance data, our ML could recommend a fitting change with a high degree of confidence, which would dramatically improve an individual’s ability to recognize human speech much better in a noisy environment. I see a huge opportunity to leverage predictive analytics and ML to significantly boost our mission of leveraging technology to empower people with hearing loss to connect with their world.
HopSkipDrive offers transportation solutions for school districts, child welfare agencies and nonprofits.
How is your team integrating AI and ML into the product development process, and what specific improvements have you seen as a result?
According to HopSkipDrive’s annual State of School Transportation report, over 90 percent of school districts suffered from a bus driver shortage in 2023, and 60 percent had reduced transportation services as a result. This is happening while chronic absenteeism is on the rise and students are still recovering from pandemic learning loss.
HopSkipDrive developed RouteWise AITM to address this problem. School district leaders determine the outcomes they hope to achieve with transportation, whether that’s navigating a labor shortage, reducing operations budgets or hitting sustainability goals, and RouteWise AI’s ML algorithm runs thousands of scenarios to find the optimal transportation plan for that objective.
Districts are seeing the opportunity for >20 percent capital and operating budget savings as well as multiple pathways for resolving their driver shortages. One district, Colorado Springs District 11, solved its driver shortage with RouteWise AI, consolidating bus routes and increasing on-time arrival rates from 85 percent to 99 percent. All of this was achieved without reducing the number of students transported and with virtually no increase in the amount of time students spent in transit.
What strategies are you employing to ensure that your systems and processes keep up with the rapid advancements in AI and ML?
RouteWise AI uses AI-powered ML to create customized routing plans tailored to each district’s specific requirements, priorities and constraints. The tool doesn’t just rearrange vehicles; it identifies opportunities to develop a multimodal transportation plan, ensuring buses are full and supplementing with the most effective arrangement of vans and small vehicles.
We’re always exploring ways to incorporate AI and ML into our workflows to boost efficiency and enhance our products, in addition to our RouteWise AI offering. When we find a potential use case, we experiment with new technologies and tools to gain practical experience and determine which ones are best suited to our needs. We involve team members from various roles, encouraging them to learn how to use AI and ML tools, while offering guidance on the most suitable platforms for their specific needs. As a company, we’re dedicated to promoting data literacy by empowering all employees to comprehend AI and ML.
“As a company, we’re dedicated to promoting data literacy by empowering all employees to comprehend AI and ML.”
Can you share some examples of how AI and ML has directly contributed to enhancing your product line or accelerating time to market?
RouteWise AI’s ML algorithm is an essential component of its competitive advantage. The current crisis has taught us that student transportation is too complex to be effectively planned and managed without the support of more sophisticated technology. Putting the power of ML at the fingertips of school district leaders and the teams that oversee transportation is charting a new path for the industry.
InspiringApps designs and develops applications for mobile phones, tablets and smart devices.
How is your team integrating AI and ML into the product development process, and what specific improvements have you seen as a result?
With 17 years of experience, we’ve seen AI and ML transform from buzzwords into household names. It would be safe to say that some aspects of these technologies have been a part of InspiringApps’ processes from the beginning. As Gen AI use cases have accelerated, our teams have also applied those tools to create efficiencies and improvement. For example, our marketing team used augmented writing in the pre-ChatGPT era. But now, we use AI tools to streamline content generation, market research and communications.
Furthermore, our design team employs AI-enhanced tools to generate connector lines and fills. From a development and QA standpoint, we’re exploring AI-assisted coding, infrastructure generation and automated testing. In project management, we’re exploring how we use AI for workflow optimization. While integrations have led to increased efficiency and time savings, we maintain a balanced approach, recognizing that practicality, security and quality remain crucial. We aim to use AI and ML to augment our team’s skills, allowing us to deliver more innovative, user-centric digital products that elevate our clients’ brands while placing data privacy at the forefront.
What strategies are you employing to ensure that your systems and processes keep up with the rapid advancements in AI and ML?
AI at InspiringApps is everyone’s responsibility, and we reflect that in reporting on our AI progress as we go. This is facilitated through regular AI-focused meetings, workshops and status updates, which provide a forum to discuss new advancements and their potential applications in our work. Through our AI at IA initiative, we ensure that everyone has a seat at the table and treat AI as a shared growth strategy.
We maintain a balanced internal and external approach, focusing on quick wins and high-impact AI opportunities. Real-world implementations allow us to test and evaluate new technologies, gauging their effectiveness and potential impact. We also proactively explore AI implementation ideas for client projects. Ethical considerations are paramount in our AI strategy. We recognize both the potential and limitations of current AI technologies, allowing us to make informed decisions about their implementation. We have an AI governance board, centralized documentation and clear policies and brand guidelines that ensure we both stay one step ahead of the latest in AI and ML while doing so responsibly in service of our clients.
“Ethical considerations are paramount in our AI strategy.”
Can you share some examples of how AI and ML has directly contributed to enhancing your product line or accelerating time to market?
AI and ML has enabled us to create more dynamic, personalized experiences within our digital products, directly contributing to increased user engagement and retention. One notable application of AI in our product line is a Gen AI solution we developed for content-heavy use cases. This tool supports users by automating various content generation tasks, saving time and enhancing the overall product experience.
AI integration has accelerated time to market for tasks and platforms with user-generated content. For instance, we built a back-end content management system that uses AI to scan and verify user-submitted content. This system employs sophisticated algorithms to detect offensive language and potential trademark infringements automatically.
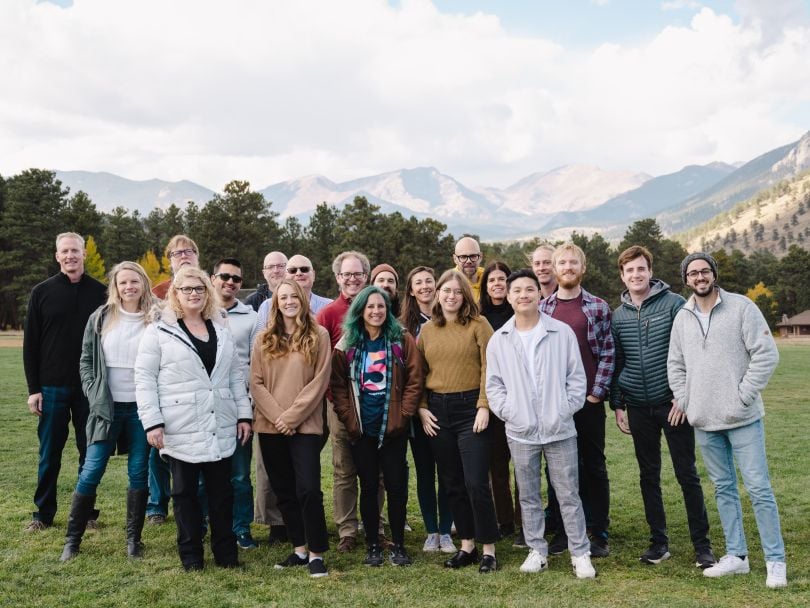
Vail Systems’ solutions help businesses make customer calls more effective through speech analytics, real-time quality feedback, automated authentication and more.
How is your team integrating AI and ML into the product development process, and what specific improvements have you seen as a result?
One example is that we’re developing ML pipelines to assist in filling out requests for proposals. Our system retrieves information from internal knowledge bases and a LLM is configured to present it in the requested format. When equipped with knowledge base results as context, the LLM can determine how our company’s capabilities align with requirements for potential projects. As a result, initial responses to many questions on these forms can be pre-filled before undergoing human review.
This initiative increases the efficiency with which employees can answer questions about company capabilities. By indexing large sets of documents, the system also provides the opportunity to incorporate relevant information that may have otherwise escaped notice.
What strategies are you employing to ensure that your systems and processes keep up with the rapid advancements in AI and ML?
We seek out new publications via literature review, attend academic conferences and industrial meetups, subscribe to AI and ML mailing lists and keep an eye on the latest projects from different companies and leaderboard sites, such as Hugging Face. The next step is thinking about how we could apply these findings to our own use cases. Our research group also hires PhD interns with diverse academic backgrounds from different universities, and we publish our results whenever possible.
Ultimately, the key aspect that keeps this system running is our team’s collaborative culture. We’re always sharing the things we discover, whether it’s in an email thread or an impromptu discussion over lunch. These exchanges often naturally spiral into brainstorming sessions that can last several days.
“We’re always sharing the things we discover, whether it’s in an email thread or an impromptu discussion over lunch.”
Can you share some examples of how AI and ML has directly contributed to enhancing your product line or accelerating time to market?
Our offerings to clients incorporate tools that extract and analyze the subjects of conversations that take place on our platform. New advances in ML have improved the quality of automated summaries and unlocked the possibility of easily answering specific questions about the data at scale. Our team is also developing solutions that can synthesize multiple sources of information in real time to provide the most helpful response during a phone call.
Some enhancements to our product line leverage tools that we’ve built in anticipation of future requirements. We’ve developed a robust speaker recognition system, which can validate the identity of a caller over the phone. This will be useful for some of our clients that want to implement additional security measures. Moreover, due to the increased risk of fraudulent callers and voice spoofing, we’ve created novel models to identify fake voices in telephonic environments. Typical customers are still in the early stages of considering this problem, but as soon as their need arises, we’ll have solutions to offer them.
Nisos provides organizations with expert consulting services meant to reduce digital risks, such as risk assessments and monitoring.
How is your team integrating AI and ML into the product development process, and what specific improvements have you seen as a result?
One of the fastest ways to get value from AI and ML is by automating repeatable use cases that traditionally consumed significant manual effort. Leveraging LLMs, we’re driving automation at unprecedented speed and scale, allowing our teams to streamline workflows and reduce human intervention. These LLMs more quickly produce tailored reports, summaries and data insights with a level of consistency and clarity that scales effortlessly across our product lines. As a result, we’ve seen marked improvements in delivery times and an increase in our capacity to handle complex, high-volume tasks — all while maintaining the human touch our customers expect.
What strategies are you employing to ensure that your systems and processes keep up with the rapid advancements in AI and ML?
We’ve implemented a modular and scalable architecture, allowing us to easily integrate new AI and ML models as they evolve without overhauling our existing infrastructure. Regular audits of our AI pipelines ensure that both the models and the data these pipelines rely on are optimized for accuracy and efficiency. Our dynamic and agile framework ensures we can innovate rapidly while maintaining stability and operational excellence.
“We’ve implemented a modular and scalable architecture, allowing us to easily integrate new AI and ML models as they evolve without overhauling our existing infrastructure.”
Can you share some examples of how AI and ML has directly contributed to enhancing your product line or accelerating time to market?
As part of our roadmap and product vision, we’re building our transformative AI and ML foundations to embed AI and ML technologies that put the power of our world-class analytic tradecraft directly into the hands of our clients. Through the use of sophisticated ML models, we will analyze massive datasets to uncover hidden patterns, emerging threats and potential vulnerabilities, providing clients with actionable insights in real time.
Our AI-powered platforms will not only automate the detection and monitoring processes, but also deliver tailored risk assessments and predictive analytics that build upon the expertise of our in-house analysts. This would empower our clients to independently navigate complex security landscapes with the insight and precision that our own teams employ, enabling faster, smarter decisions.
Kalderos’ platform helps enterprises manage drug discount programs.
How is your team integrating AI and ML into the product development process, and what specific improvements have you seen as a result?
We use Gen AI for internal and operational productivity, and GitHub CoPilot is one of the key accelerants for not only developing code, but also tests. We’ve seen productivity gains in development and testing speed as a result of these efforts.
What strategies are you employing to ensure that your systems and processes keep up with the rapid advancements in AI and ML?
Kalderos ensures that our teams have access to learning platforms to keep up with and adopt advancements in AI and ML. We have an AI policy that helps our teams use advancements in a safe and secure way, poised to evolve with the AI landscape to take on future challenges within this market.
“Kalderos ensures that our teams have access to learning platforms to keep up with and adopt advancements in AI and ML.”
Can you share some examples of how AI and ML has directly contributed to enhancing your product line or accelerating time to market?
Kalderos has been on the AI journey nearly since its inception. As a company trying to create transparency in the drug discounts space where data and insight visibility is key, AI and ML play a key role in filling some of the gaps and are used in our product suite to identify non-compliance and drive better outcomes for our customers. Our products are built on a strong data foundation, which allows us to take advantage of AI in a significant way for both internal and customer use cases.