The so-called “superforecasters” started contemplating how COVID-19 might unfold across the United States back in early April. It was still the early days of the pandemic in the States, with quite a bit of disagreement among the various models, and data so messy that some simply chose not to predict. But messy-data, high-interest questions are precisely what superforecasters aim to clarify.
To properly tackle the overall picture, they broke it down into several questions. Taken in isolation, any single one might sound like a morbid parlor game, but the goal was to better understand the situation, not glibly speculate.
“Folks were all over the map on what it was that had hit us hard a month earlier,” said Marc Koehler, vice president of Good Judgment and a superforecaster himself.
One of the questions they considered in the difficult process: How many people in California will have died from COVID-19 by the end of June?
Like all non-yes/no questions that superforecasters consider, the question was framed in a multiple choice format, with each of the five options in this instance listed as a numerical range. They chose the option that ranged between 3,900 and 19,000 deaths.
But superforecasters — the cream of the crop of predictors affiliated with the Good Judgement project — don’t simply vote yes or no, they assign probabilities, then adjust them as time goes on and variables change. Before April was over, the group had assigned their range a 50 percent probability. They were already confident in their selection early on.
“And [the probability] just went up from there,” Koehler said. Unfortunately, the high-end estimate was spot-on. There were 6,082 COVID-19 deaths in California by June 30, according to Johns Hopkins University’s Coronavirus Resource Center.
Sure, a range of 15,000-plus isn’t exactly hyper-specific, and only five possible choices is pretty good odds. And unless you’ve assigned a probability of 100 percent, an outcome in itself doesn’t prove or disprove a forecast’s quality, as post-2016 Nate Silver is surely very tired of explaining.
But especially given the high degree of uncertainty at the time, Koehler pinpoints the prediction as a recent example of superforecaster success, if one we all wish they had overestimated. The example also gets at Good Judgment’s approach of giving equal, if not greater footing, to psychology alongside the data science that traditionally drives predictive analytics.
Here’s Koehler’s working hypothesis: “Modeling is a very good way to explain how a virus will move through an unconstrained herd. But when you begin to put in constraints” — mask mandates, stay-at-home orders, social distancing — “and then the herd has agency whether they’re going to comply, at that point, human forecasters who are very smart and have read through the models, that’s where they really begin to add value.”
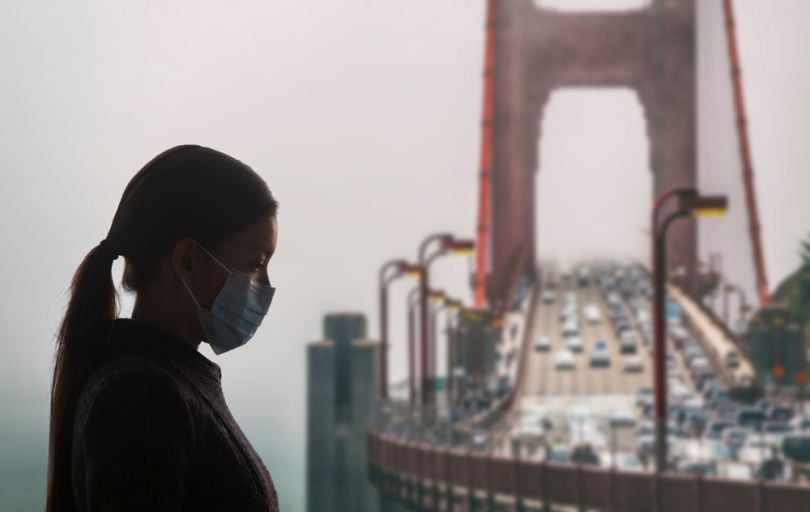
A Brief History of Good Judgment
Good Judgment has made headlines recently, thanks to COVID-19 predictions and some high-profile co-signs, but its origins date back decades. University of Pennsylvania psychologist Philip Tetlock in 1984 started hosting small forecasting tournaments, inviting more than 250 people whose professions centered around “commenting or offering advice on political and economic trends,” according to Tetlock’s 2005 book Expert Political Judgment.
By 2003, the group of pundits had made tens of thousands of predictions. Their track record turned out to be ... not great. In fact, they would have performed better had they simply assigned each potential outcome, no matter how seemingly unlikely, an equal probability. As Tetlock put it, they fared little better than “dart-throwing chimps.”
If those findings prompt more anxiety than they do proletarian exhilaration, given our regrettably anti-expert era, Tetlock feels your pain. He lamented in the book’s 2017 edition that “the media misinterpreted EPJ to claim that experts know nothing, and know-nothings seized on that claim as proof that knowledge itself is somehow useless.”
The better lesson, according to Tetlock, is that domain expertise and predictive ability are not necessarily correlative.
The U.S. intelligence community took notice of Tetlock’s research and started staging its own forecasting tournaments in 2011, with Tetlock’s cohort in tow. The Intelligence Advanced Research Projects Activity challenged participants to develop better ways of recruiting and training forecasters and aggregating their results. The insights Tetlock gleaned — working in teams improves forecasts, as does a readiness to admit error, an actively open mind, and extremizing forecasts in the aggregate — would come to form the basis of Good Judgment.
What Makes a Good Forecaster?
In his 2015 book Superforecasting, Tetlock expounds at length about the characteristics, and sometimes paradoxes, that generally define superforecasters. For instance, several have advanced math or science degrees, but most don’t use a ton of math in their process. When I asked Koehler (left) for a CliffsNotes version of what makes a superforecaster super, he pointed to three key traits.
Interestingly, people who perform well with pattern recognition, particularly on Raven’s Progressive Matrices test, tend to be more accurate forecasters, he said. There’s also the humility aspect, which comes up often in Superforecasting — but it’s a bit more rigorous than just admit when you’re wrong. The best forecasters often utilize a framework known as actively open-minded thinking. The concept, coined by psychologist Jonathan Baron, a colleague of Tetlock at Penn, encourages actively seeking out reasons why one might be wrong, not simply being open to consider them.
“Will we double down on the judgment we made or would we be willing to completely reverse course and head in another direction if the information warranted?” Koehler said. “People who do that tend to be better forecasters.”
“People who update their forecasts by little tiny steps are more accurate than people who sort of fire and forget.”
The third facet is an emphasis on what Koehler calls cognitively reflective thinking. In the simplest terms, it means making a habit of interrogating your gut feelings.
“When you’re asked to make a judgment, an answer will often suggest itself to you,” he said. “Some people stop and check their thinking. ‘This popped in as the right answer; is it in fact the right answer?’ That correlates with forecasting accuracy. It’s part nature and part nurture,” he said.
Good Judgment offers a variety of free resources forecasters can use to train these three muscles. It also offers the training ground. The team in 2015 launched Good Judgment Open, a public prediction market where all are invited to weigh in on questions ranging from “How many states will have reported more total COVID-19 cases for September 2020 than for June 2020?” to “Will the federal emergency increase in unemployment compensation benefits be extended before 1 August 2020?”
It’s not dissimilar to betting markets like PredictIt or Metaculus, except GJO also acts as a farm system, with the best performers getting call-up offers to the “super” leagues.
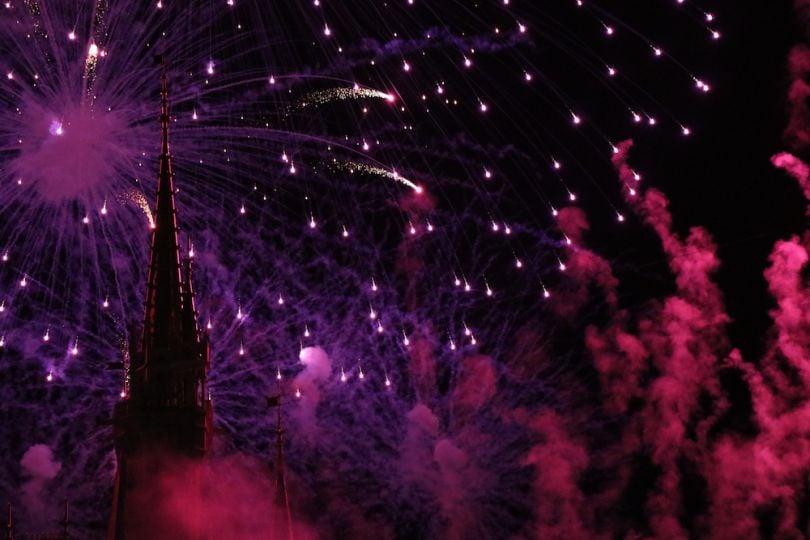
Enter the Algorithm
The characteristics laid out above are all squarely in the psychological realm, which might suggest a sidelining of statistics and data science. And Tetlock does indeed paint a curious math-people-who-shun-math paradox among top-flight forecasters.
Perhaps the most striking example is Cornell University math professor and superforecaster Lionel Levine, who, “in a contrarian way,” aimed to prove his forecasting chops “without using any [math],” Levine is quoted as saying in Superforecasting. There’s also Tim Minto (no longer an active superforecaster), who “knows Bayes’ theorem but didn’t use it even once to make his hundreds of updated forecasts,” Tetlock wrote. “And yet Minto appreciates the Bayesian spirit.”
But don’t be misled. Good Judgment incorporates data analytics expertise in some key ways, sometimes in individual forecasts, but perhaps most notably in GJ’s aggregation algorithms.
Be they from finance, energy, government or some other sector, when a client comes to Good Judgment with a question it wants considered, they encounter a formalized process. First, there’s an extensive process of hammering down and framing the question. Then the call for superforecasters goes out. (Each task is assigned a group of about 40 superforecasters.)
“And then they start forecasting,” Koehler said. “And people who update their forecasts by little tiny steps are more accurate than people who sort of fire and forget.”
Some superforecasters also build their own models. Indeed, a number of superforecasters are professional data scientists, according to employment-board profiles. “Some will build a computer simulation or do a Bayesian decision tree,” Koehler offered.
Then, after several days of analysis and testing the question framing, Good Judgment uses its in-house algorithms to aggregate the team’s probabilities. “The nice thing is that any errors tend to be randomly distributed,” he added. “They’ll be left and right of truth and when we aggregate all the judgments together, your overestimate and my underestimate tend to cancel each other out.”
The aggregated forecasts are then updated daily.
Good Judgment has done some notable work on this particular front. A 2013 paper co-authored by Good Judgment co-founders outlined a formula for combining multiple probability forecasts that was “found to be superior to several widely used aggregation algorithms.” Even though self-purported experts are often overconfident, they are underconfident as a group. “Therefore, if no bias-correction is performed, the consensus probability forecast can turn out to be biased and sub-optimal in terms of forecast performance,” they wrote.
“Your overestimate and my underestimate tend to cancel each other out.”
They followed that up with research that showed the value of weighting recent opinions more strongly than older ones, weighting opinions based on prior success, and extremizing the aggregate in order to cut down distortion. Perhaps even more significant, they found that extending such weighted aggregate algorithms to polls and surveys allows them to perform just as well betting markets. A hybrid of a prediction market and poll aggregate, meanwhile, performed better than either alone — which could prove major.
As Kelsey Piper wrote in Vox last year, “A lot of the legal and logistical barriers to prediction markets will go away if prediction aggregators that don’t involve betting money can achieve the same thing.”
Are Hybrids the Future of Futurecasting?
Of course, the quest for a better crystal ball doesn’t stop at even advanced aggregation techniques. The IARPA contests where Tetlock’s ideas came of age have now evolved into so-called hybrid forecasting competitions, where the goal is to build systems that combine human and machine learning forecasting components “to create maximally accurate, flexible, and scalable forecasting capabilities.”
So how are they performing? That’s not yet fully clear, although at least one participant says its hybrid approach is producing better Brier scores — the metric by which probability forecasts are graded — than human-only forecast control groups.
Still, Tetlock and Koehler both sound skeptical as to how much machine learning models will help predict the kinds of questions that make up Good Judgment’s bailiwick — low- or messy-data questions that we all still really want answers for.
“The things like the Syrian Civil War, Russia/Ukraine, settlement on Mars — these are events for which base rates are elusive,” Tetlock said on a recent podcast appearance. “It’s not like you’re screening credit card applicants for Visa. Machine intelligence is going to dominate human intelligence totally. Machine intelligence dominates humans in Go and Chess. It may not dominate humans in poker.”
The models “still have some distance to travel before they can correctly model what humans are going to do in complicated situations, it seems,” Koehler said.
So while the present nature of forecasting is a complex melange of psychology, social science and data aggregation algorithms, the future of prediction is not entirely known.