In just five days, ChatGPT accomplished something that took Twitter two years to do — reach over 1 million users. Generative AI set a precedent in the tech industry, and it’s growing faster than most teams can keep up.
“With GPT technology we can finally reach that high bar,” said Diana Mingels, head of machine learning at Kensho. “Those companies who haven’t started integrating it yet are already behind.”
Kensho, the AI accelerator for S&P Global, is definitely not falling behind.
“Those companies who haven’t started integrating [generative AI] yet are already behind.”
The company’s product team develops AI tools that can tap into the reservoir of S&P’s big data to inform users on complex financial questions.
The sheer amount of data that S&P has access to is staggering. S&P Capital IQ analyzes more than 135 billion data points. When customers need to sort through that much data, it’s challenging. The machine learning team at Kensho started to wonder how they could make the process of understanding that data more accessible for their customers — which is how the idea for a new generative AI tool, ChatIQ, was born.
“Due to the ChatGPT craze earlier in the year, there were high expectations on what a generative AI tool could do,” said Lupe Carino, product manager at Kensho. “We aim to create a product that transforms and adds value to the way clients interact with data to answer complex financial questions.”
However, the anticipation and excitement the team felt about creating a generative AI product was not without reservations.
“My biggest concern with generative AI early on was how to deal with veracity,” said Zak Brown, machine learning manager of natural language processing at Kensho. “Large language models are not incentivized to produce factually correct information, and that’s problematic when your business is largely dealing with factual, and often quantitative, information.”
Ensuring that generative AI presents accurate information is one of the biggest setbacks that keeps it from competing with search engines just yet. The issue with a model like ChatGPT is that it only responds by using the data it trained on. Wired notes that retraining an AI model can cost “millions of dollars because of its size and the scale of the data.”
There is a sweet spot, though — one where the foundational data is accurately training the generative AI. This Goldilocks zone for generative AI is right within Kensho’s reach, bringing together accurate information and massive data sets.
“Kensho holds a very unique place in the industry in that we have the agility of a startup, but access to a scale of data assets that typically only exist in larger enterprises,” Brown said. “Because of this, we’re able to work at the bleeding edge of machine learning research and development and build systems that are trained and interact with massive data stores.”
“Kensho holds a very unique place in the industry in that we have the agility of a startup, but access to a scale of data assets that typically only exist in larger enterprises.”
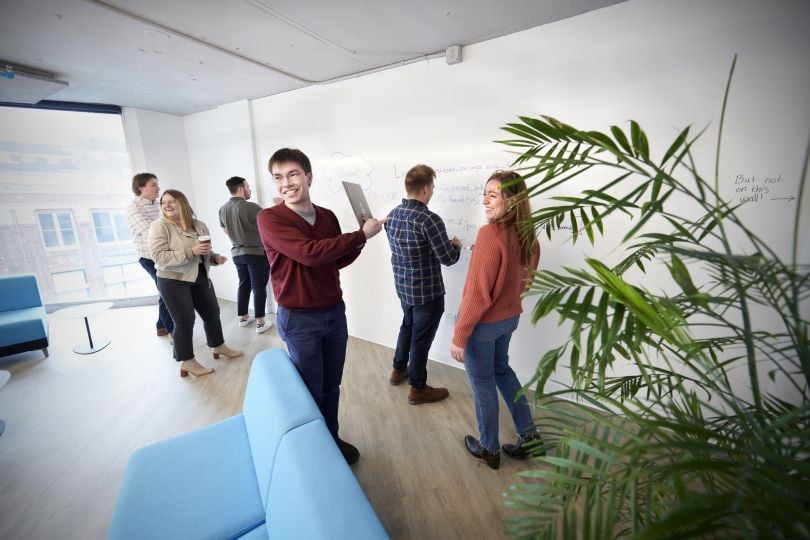
Solving the Generative AI Problem
The machine learning team at Kensho is not only creating a new level of user simplicity, it is completely changing the way its generative AI tool sources information, which is an ongoing problem for most who work in AI.
The problem is fairly simple, but a hard one to fix. Say a user decides to ask a generative AI chatbot, like ChatGPT, a question instead of searching for the answer on their own. At the moment, most generative AI chatbots are a bit of a gamble when it comes to getting an accurate response. It’s a little like asking a librarian what section of the library has books on classic art and having them respond back with the location of the Mona Lisa — it might relate to the question, but it’s definitely not the right answer.
“My biggest ‘aha’ moment was when I realized the power of these models in use cases involving retrieval and generation,” Brown explained, referencing Kensho’s access to S&P Global data.
S&P Global accesses over 124,000 global public and private companies worldwide with its financial data, according to NYU’s library research guide.
“With that type of framing, we can pin any information returned from a model to underlying factual data sources,” Brown continued. “There’s always a path to value for machine learning use cases — with some paths wider than others. With that realization it became clear to me that the value path for large language models for S&P was a 12-lane superhighway that was wide open for us.”
“It became clear to me that the value path for large language models for S&P was a 12-lane superhighway that was wide open for us.”
What sets ChatIQ apart from other generative AI tools is its powerful data training and ease of use for customers. With the tool, users are able to pull information from a multitude of data sources using only natural language. Previously, they would navigate an information-rich dashboard-like environment — now, they experience a clean, modern interface where they can ask questions and receive rich, contextualized answers.
“[ChatIQ] will significantly reduce client time required for navigating, searching, discovering and creating on the CapIQ Pro platform,” Carino said.
Mingels notes how meaningful well-functioning generative AI really is. “The human-to-human quality interactions of the pre-digital era are now coming back in a new form of human-to-computer interactions,” Mingels said, noting how generative AI needs the fluidity of a conversation. “This is truly remarkable!”
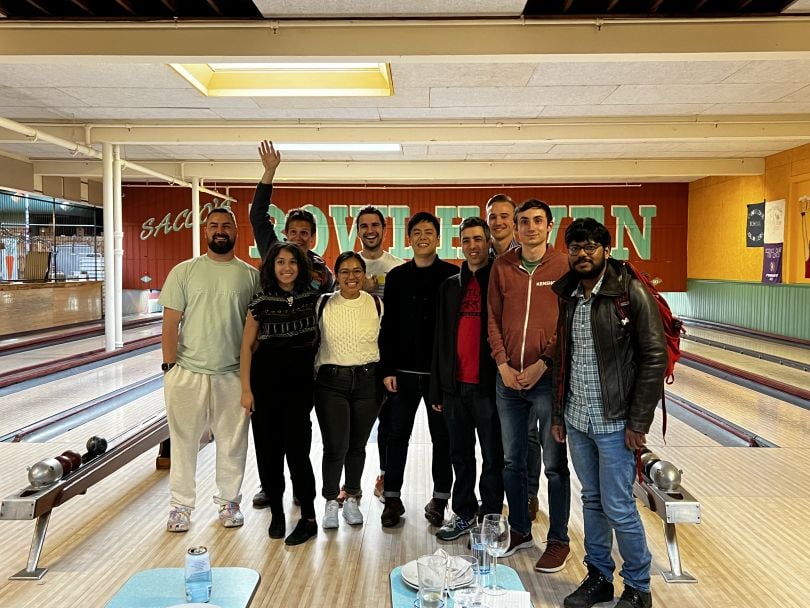
Flexibility is True North for Product Design
The pressure was on for the Kensho team when the ChatIQ quest launched. “For Kensho, we have a fair amount of flexibility in terms of what we can build and how often we can deploy,” Carino said. “This is important because it allows us to learn and iterate quickly. Our broader company, S&P, does not have the same level of flexibility as we do because they are deploying large enterprise software. Instead, they deploy on a much longer timeline.”
The team definitely had to be quick on their feet. In under eight weeks they were able to produce a minimum viable product. Carino spent much of those eight weeks guiding the team on which decisions could be made immediately and which required more thoughtfulness. “Prioritization was also important because there was only so much functionality we were able to build in a given time,” said Carino.
“As the Generative AI technology is very new, there are a lot of solutions and businesses emerging around making it accessible, scalable and easy to adopt,” Mingels said. “However, many of those solutions are either not enterprise-grade or very rigid and opinionated.”
Mingels explains how, in order to move quickly, her team built ChatIQ with in-house tools and custom implementations. “As the product evolves, we are adopting some of the third-party models and packages that are becoming more stable, secure and popular. We will continue evaluating different solutions and approaches while combining them with our in-house expertise as we move forward.”
The Kensho team is now in a position to scale ChatIQ very quickly. “We’re leveraging the latest advancements in generative AI to power ChatIQ, finding new and useful applications nearly every day,” Brown said. “Using these technologies, we’re able to build flexible, responsive systems in a way that wouldn’t have been possible several years ago.”
Brown has worked in conversational AI and information retrieval spaces for several years and notes how far the field has come in just a short period of time. “[It’s] evolved from fine-tuned yet brittle systems to truly dynamic systems that can react to a multitude of use cases.”
“It is a large undertaking,” Carino added, noting the amount of ground already covered. “There are data retrieval, data ingestion, security, speed, entitlement issues and more to consistently think about, on top of figuring out how to build the best tool that addresses key business and finance use cases. Nevertheless, we’re here for the challenge.”