Sometimes a ratio just works. The so-called golden ratio, or divine proportion, repeats again and again in nature — popping up everywhere from flower petals to pine cone spirals. In tech teams, we haven’t found anything quite so cosmically aligned, but the search for balance remains an evergreen concern. Consider the question: What’s the best ratio of junior-to-senior contributors?
It’s an oft-discussed topic in software engineering, but less so in data science. There is, of course, best-practice advice around team construction for data teams, forever evolving though it may be. But that tends to revolve around considerations like the number of data engineers per data scientist. As dbt Labs CEO and founder Tristan Handy noted earlier this year, the junior-to-senior consideration is more of an open issue for data teams.
“It’s a bit of a balancing act.”
Still, it’s worth considering seriously. Lyndsey Padden, vice president of data science at 84.51°, a Kroger-affiliated marketing analytics firm, told Built In that failing to maintain a proper balance can either stifle professional development or diminish the overall quality of work.
“[Having] too many senior data scientists creates a top-heavy organization and doesn't necessarily give people the right accountabilities or opportunity to stretch into talent management — or even technical skill development areas,” said Padden.
On the other hand, “if you have too many juniors, you don’t necessarily have the right support system in place,” she added. “Or you aren’t giving your senior data scientists enough room for strategic thought leadership” and ample command over workflows. “So it’s a bit of a balancing act.”
So what is the ideal ratio, assuming one even exists? And how important is maintaining it?
How Are the Roles and Responsibilities Allocated?
Before we explore the numbers, let’s dissect what we mean by junior, mid-level and senior data scientists beyond simply time in industry. Having clear a definition and distribution of responsibilities goes a long way when thinking about staffing.
Below is a chart of how data science teams approach task management, broken down by seniority level, according to staffing research published last year by Gartner. Junior core responsibilities include preparing and refining data (including feature engineering), exploring and understanding data, and building machine learning models. Senior core tasks, meanwhile, include guiding and storytelling — that is, pinpointing modeling opportunities that realistically dovetail with larger business focuses — and formulating and prioritizing projects.
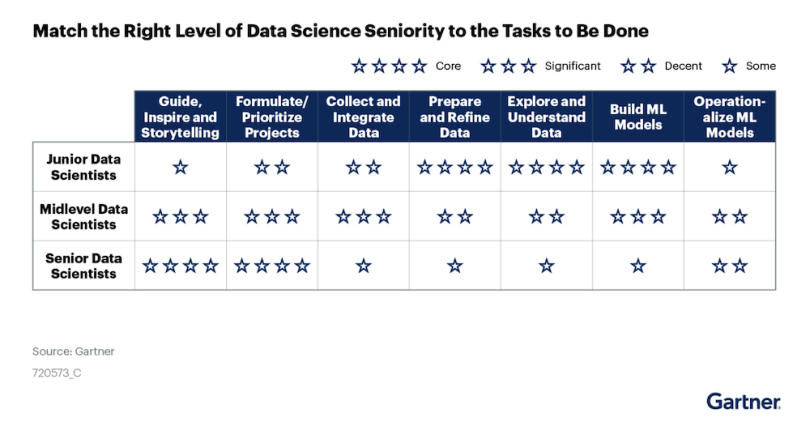
Maureen Kalas, director of data, discovery and decision sciences at Allstate, said that Gartner’s breakdown roughly accords with how her company operates, including prioritizing data prep, but also model construction, for juniors.
“We expect our junior folks to be building models,” she said. “It’s not really very fun for them if they are coming in and only fooling around with getting data clean.”
That said, even though candidates tend to arrive directly from bootcamp or university programs with strong understandings of how to build models in a sandbox environment, learning when and where to apply that knowledge in the real world often takes time.
“You may be excited to build a model to predict this, that and the other, which you’ve simulated in a classroom environment,” said Padden, of 84.51°. “But until you’ve had an opportunity to come in and ask, ‘Is that actually needed to solve this business problem and what’s the best way to do that?’ — that’s where some seniority and professional experience comes into play.”
At Allstate, mid-level data scientists interact more with other departments within the company to help clearly define the issue at hand.
For instance, when the company recently updated its advanced analytics function for human resources, it was mid-level data scientists working with HR to initiate the work. In addition to big-picture vision, seniors at Allstate also look for automation opportunities and often focus on maintaining models in production by watching for drift.
“It’s a broader and wider accountability,” said Kalas.
My Ideal Ratio Might Differ From Your Ratio
At 84.51°, the ratio has historically been somewhere between three or four junior data scientists to one senior data scientist, according to Padden. As the organization has matured, it’s tried to keep the ratio closer to 3-to-1.
Having fewer direct reports means senior data scientists can continue to develop their technical and roadmapping skills, and not become wholly devoted to people management, she said. But an entire team of senior-level talent — in addition to being expensive — poses growth and advancement problems.
“Having too many seniors can limit development opportunities for people in that space, too,” Padden said. “It’s beneficial for folks on a director track to have talent they can coach, grow and develop.”
While 84.51° appears to have found its number, Kalas, at Allstate, is content to allow the proportion to fluctuate as it may. Right now, the insurance firm’s junior-to-senior ratio in data science is roughly 1-to-1, but factors like growth strategy and the nature of the talent landscape have impacted that figure in the past, and may do so again in the future.
About four years ago, for example, Kalas oversaw a team that had more junior talent, but that was in part because the organization was undertaking a big hiring push and the prospect pool back then simply had more entry-level prospects than advanced candidates.
“At that time, the supply of data scientists was pretty small, relatively speaking,” Kalas said. “That has improved significantly over the years, as schools are churning out more people. … Now there are more seniors out there.” In the past, it was easier to find candidates with two or fewer years of experience “and train them up,” she added. “Now we can bring in a mix.”
Allstate is now in the midst of another data science hiring push and however the mix of experience levels shakes out afterward won’t be strictly predetermined. There’s no target ratio and Kalas isn’t sure a “proper” ratio exists, “at least for us.” This isn’t to say they’re only pushing one way or the other.
“Having a balance — a mix of juniors and seniors — helps ensure that you've got a good pool of leaders who can organizationally manage, but also provide a lot of technical guidance,” she said.
It’s More Important to Keep the Pipeline Humming
Opinions may differ as to whether a set junior-to-senior ratio is worth maintaining, but a related item is probably universal: It’s important to keep the pipelines to either level running smoothly. Kalas and Padden both stressed the importance of nurturing talent, regardless of experience level, and making sure all have room to advance.
To that end, at 84.51°, the data science department offers a three-month internship. Growth projections dictate how many interns are offered full-time positions each year, but conversion is reliably “strong,” Padden said. The firm also partners with universities to attract entry-level staffers each autumn.
“The freedom to grow at your own pace and have opportunities for deep learning — that’s critically important and it ends up influencing what a ratio looks like.”
For higher-level positions, there’s a so-called technical track, which allows senior-level data scientists to still spend significant time doing technical work. Roughly 30 percent of senior data scientists are on the technical track, Padden said.
Kalas mentioned an effort at Allstate, coming later this year, that will target candidates from underrepresented backgrounds who didn’t have access to graduate programs. Those who are hired will undergo a thorough rotational training process that’s intended to shore up any data science skill gaps. All new data science hires are also given an onboarding project, lasting between two to four months, before “graduating into working on their own.”
“The freedom to grow at your own pace and have opportunities for deep learning — that’s critically important and it ends up influencing what a ratio looks like,” Kalas said.